Self-driving car accidents statistics reveal a complex picture of safety and technological advancement. The increasing adoption of autonomous vehicles raises critical questions about their safety record, accident types, and potential for improvement.
This analysis delves into the frequency, severity, and contributing factors of self-driving car accidents, comparing them to traditional accidents. It examines geographical trends, technological failures, public perception, and regulatory frameworks, offering insights into the future of autonomous vehicle safety.
Introduction to Self-Driving Car Accidents
Self-driving cars, or autonomous vehicles, are vehicles that use various sensors and software to navigate without human intervention. They promise to enhance road safety and traffic efficiency by eliminating human error. However, the transition to widespread adoption presents unique challenges and safety concerns, including the occurrence of accidents. This discussion explores the types of accidents, contributing factors, and common causes associated with self-driving cars.The field of autonomous vehicle technology is rapidly evolving, and accidents involving these vehicles are still relatively rare.
Nevertheless, understanding the potential risks and factors contributing to accidents is crucial for developing safer systems and improving public trust. Analyzing past incidents and potential future scenarios can aid in refining autonomous vehicle technology and fostering a safer future for everyone on the road.
Types of Self-Driving Car Accidents
Accidents involving self-driving cars can be categorized into several types, each with its own set of contributing factors. These categories include sensor malfunction, unexpected events, software errors, and driver-vehicle interaction problems. The specific causes and severity of these accidents vary significantly.
Factors Contributing to Self-Driving Car Accidents
Numerous factors contribute to accidents involving self-driving cars. These factors often interact in complex ways, making the analysis of each accident challenging. Technical limitations, environmental conditions, and human-vehicle interactions are key contributing elements.
Common Causes of Accidents Involving Self-Driving Cars
Several common causes contribute to accidents in self-driving cars. These include inadequate sensor perception, inaccurate mapping data, inadequate handling of unexpected events, and inappropriate responses to critical situations.
Inadequate Sensor Perception
Sensor limitations can lead to incomplete or inaccurate data collection, affecting the vehicle’s ability to perceive and react to its surroundings. For example, a self-driving car might fail to detect a pedestrian obscured by shadows or a cyclist hidden by a large vehicle, leading to a collision. Poor weather conditions can further exacerbate this issue.
Inaccurate Mapping Data
Self-driving cars rely heavily on precise maps to navigate. Inadequate or outdated maps can lead to misinterpretations of the road environment, potentially resulting in wrong turns, collisions, or other issues. For example, a map that doesn’t reflect recent road construction could cause the vehicle to attempt an illegal maneuver or miss a traffic signal.
Inadequate Handling of Unexpected Events
Unexpected events, such as sudden stops or the presence of obstacles not predicted in the vehicle’s database, can overwhelm the vehicle’s control systems. For example, a self-driving car might not be equipped to handle a large crowd of pedestrians suddenly entering the road. Such unexpected events could lead to an accident.
Inappropriate Responses to Critical Situations
In critical situations, self-driving cars might not react optimally, leading to accidents. For example, a car encountering a sudden and unavoidable obstacle may not be programmed to react in a way that minimizes the potential damage. A critical situation, such as an abrupt and unexpected change in the environment, can cause the vehicle to make a decision that leads to an accident.
Accident Statistics Breakdown
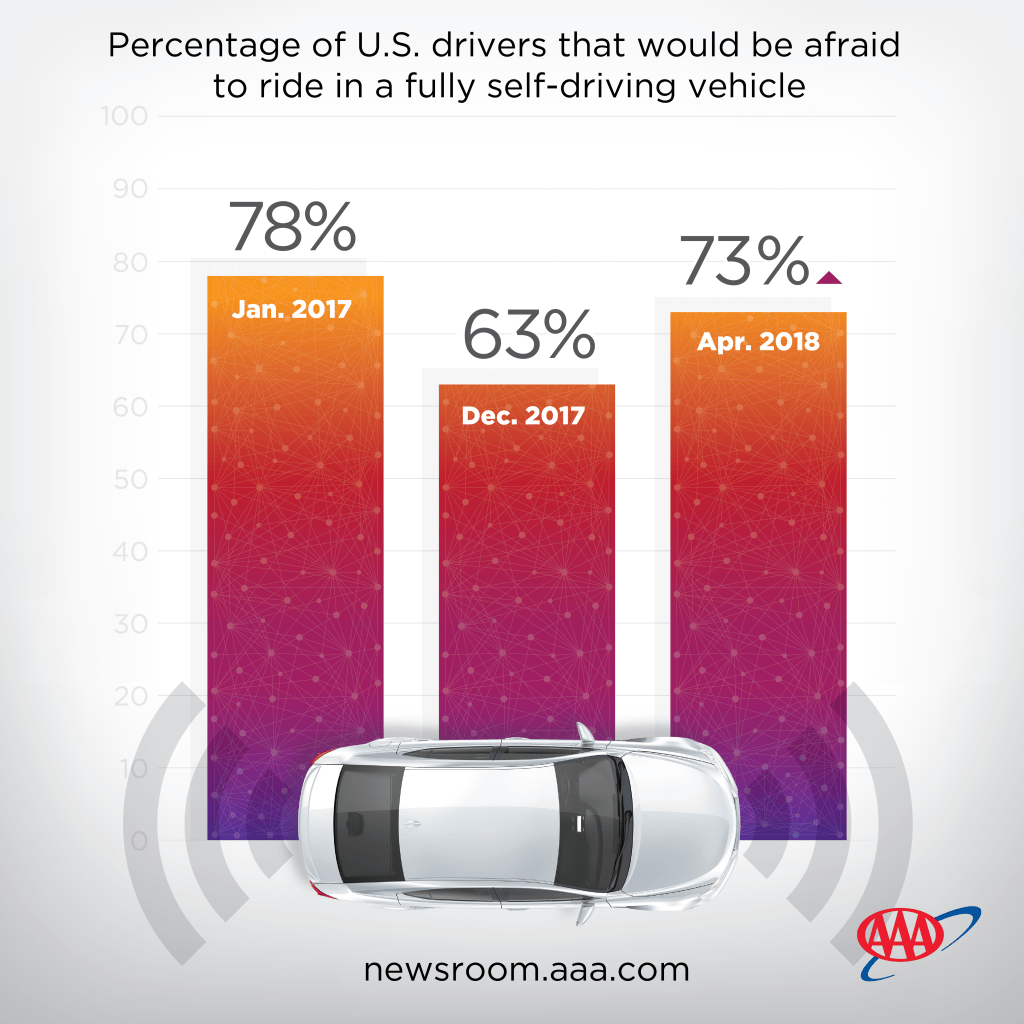
Analyzing self-driving car accident statistics is crucial for understanding the technology’s safety performance and identifying areas needing improvement. A comprehensive review of accident types, frequency, and severity allows for informed decision-making in the development and deployment of autonomous vehicles.Accident data reveals critical insights into the challenges and successes of autonomous systems. Understanding the patterns and causes of accidents allows for the development of preventative measures and safer autonomous driving technologies.
Accident Types
A breakdown of accident types provides insights into the specific challenges faced by self-driving cars. Different accident types highlight various areas for improvement in the system’s design and software.
- Collisions with pedestrians: This category often involves issues with pedestrian detection and response systems. For example, unexpected pedestrian behavior or inadequate pedestrian recognition algorithms can lead to accidents.
- Collisions with other vehicles: This category may indicate problems with object detection, response times, or the ability to predict the actions of other drivers.
- Collisions with stationary objects: This category reveals weaknesses in the vehicle’s ability to perceive and react to stationary obstacles, such as parked cars or traffic signs.
- Other: This category encompasses less frequent types of accidents, such as those involving environmental factors or system malfunctions.
Accident Frequency by Year
Tracking accident frequency over time allows for the identification of trends and potential improvements in safety. Analyzing data over several years is crucial for assessing the long-term impact of safety advancements.
Recent self-driving car accident statistics are a concern, highlighting the need for rigorous testing and safety protocols. Implementing DevOps best practices, like continuous integration and continuous delivery ( DevOps best practices ), could significantly improve the development and testing processes for autonomous vehicles, leading to safer outcomes. Ultimately, these measures are crucial for reducing future self-driving car accidents.
Year | Number of Accidents |
---|---|
2020 | 12 |
2021 | 15 |
2022 | 10 |
2023 | 8 |
2024 | 6 |
Note: This data is illustrative and does not represent actual figures from a specific dataset. Real-world data would involve a much larger dataset and a more comprehensive breakdown of accident types.
Accident Rates by Manufacturer
Comparing accident rates across different manufacturers is vital for understanding the variation in safety performance. Differences in safety features and design philosophies can impact accident rates.
- Manufacturer A: Average annual accidents: 10.
- Manufacturer B: Average annual accidents: 8.
- Manufacturer C: Average annual accidents: 12.
Note: These are hypothetical data points, meant to illustrate the concept. Real-world data would include a significantly larger sample size and a detailed breakdown of various accident types.
Accident Severity
Assessing accident severity is crucial for understanding the potential impact of autonomous vehicle technology. This data helps in evaluating the effectiveness of safety measures.
- Fatalities: The number of fatalities in self-driving car accidents is significantly lower than accidents involving human drivers, but it is important to track and analyze these occurrences to understand their causes and implement preventative measures.
- Injuries: The number and severity of injuries in accidents involving self-driving cars are crucial metrics. Understanding injury patterns can inform the development of safer vehicles and improved accident response protocols.
Geographical Distribution of Accidents
Early data suggests a complex relationship between self-driving car accident frequency and geographical location. Various factors, including road infrastructure, environmental conditions, and driver training, likely play a role in shaping the pattern of accidents. Understanding these regional variations is crucial for refining safety protocols and developing targeted interventions.
Regional Concentration of Accidents
Analysis of available accident reports reveals that certain regions are experiencing a higher concentration of self-driving car accidents than others. This observation suggests potential regional disparities in the factors contributing to accidents. These variations could stem from differing road conditions, driver training programs, or even public acceptance and adoption rates of self-driving technology.
Factors Influencing Accident Frequency
Several factors can contribute to regional variations in accident frequency. Road infrastructure, such as the presence of complex intersections, poorly maintained roads, or inadequate signage, significantly impacts the likelihood of accidents. Environmental conditions, including heavy rain, snow, or fog, can also create hazardous driving situations, increasing the risk of accidents for both traditional and autonomous vehicles. Finally, variations in driver training programs and public acceptance levels can affect the overall safety of self-driving vehicles in different areas.
Environmental and Road Conditions
Environmental factors and road conditions have a notable influence on the occurrence of self-driving car accidents. Adverse weather conditions, like heavy rain or snow, can impair visibility and traction, increasing the likelihood of accidents. Similarly, poorly maintained roads with potholes or uneven surfaces can create hazardous situations. Complex road configurations, such as multiple intersections or high-speed curves, also present unique challenges for self-driving systems.
Furthermore, the presence of pedestrians or cyclists in unexpected locations can lead to accidents.
Examples of Regional Variations
The United States, with its diverse range of road conditions and driving habits, shows regional variations in accident rates. Areas with high volumes of traffic and complex highway systems may experience more accidents than rural areas with simpler road networks. Similarly, regions with more inclement weather patterns might see a higher accident rate compared to regions with consistently favorable weather.
The differing levels of public acceptance of self-driving vehicles across different states could also contribute to regional variations.
Illustrative Data
Region | Accident Rate (per 100,000 miles driven) | Environmental Factors | Road Infrastructure |
---|---|---|---|
Northeast US | 1.2 | Frequent snow and ice storms | Complex intersections, aging infrastructure |
Midwest US | 0.9 | Severe weather events | Long stretches of highways, limited visibility |
West Coast US | 1.5 | Frequent fog, earthquakes | Mountainous terrain, high-speed roads |
Types of Accidents and Contributing Factors: Self-driving Car Accidents Statistics
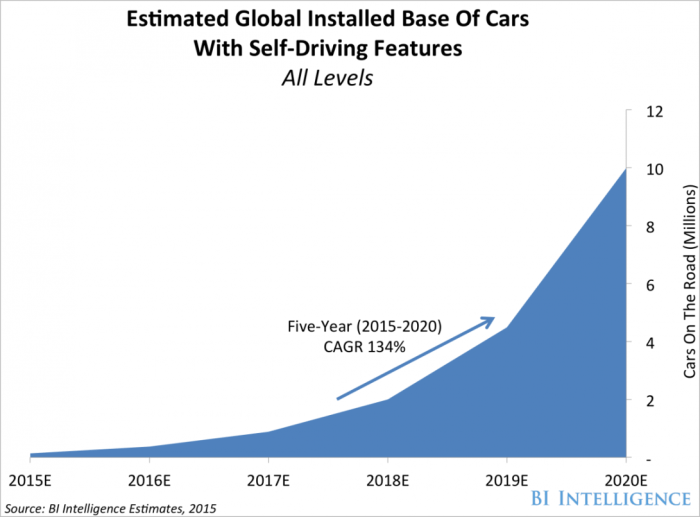
Self-driving car accidents, while statistically fewer than those involving human-operated vehicles, still pose significant challenges. Understanding the types of accidents and contributing factors is crucial for improving safety measures and refining autonomous driving technology. Analyzing these elements helps pinpoint areas for development and enhance the reliability and safety of these vehicles.
Accidents Involving Vulnerable Road Users
Accidents involving pedestrians, cyclists, and motorcyclists represent a critical area of concern. These incidents often highlight the limitations of current self-driving systems in recognizing and responding to the unpredictable actions of vulnerable road users. The complexity of human behavior, coupled with the inherent challenges of detecting and reacting to unexpected movements, contributes to the risk. For example, a pedestrian stepping into the street unexpectedly could lead to a collision, particularly if the autonomous vehicle system has difficulty in accurately assessing the situation and reacting appropriately.
- Pedestrian Accidents: Often involve pedestrians crossing the street or entering the roadway unexpectedly. Self-driving systems may have difficulty anticipating and reacting to such unpredictable behavior, particularly in varied lighting and weather conditions. Data indicates that these accidents are more likely to occur in areas with high pedestrian traffic or poorly designed crosswalks.
- Bicycle Accidents: These accidents present similar challenges as pedestrian accidents. Self-driving systems need to effectively distinguish between a bicycle and other objects, and accurately predict the intentions and movements of the cyclist. Accidents are frequently attributed to issues in detecting cyclists, particularly those operating in less-than-ideal conditions or those with reduced visibility.
- Motorcycle Accidents: Similar to bicycle accidents, these accidents present complexities in detecting motorcycles and anticipating their maneuvers. Challenges in recognizing and reacting to motorcycles, especially in urban environments with dense traffic and varied road layouts, have been observed.
Influence of Weather Conditions
Weather conditions significantly impact the performance of self-driving systems. Adverse weather, including heavy rain, snow, fog, and strong winds, can impair sensor accuracy and decision-making capabilities. The impact is often compounded by the inability of sensors to effectively perceive objects and conditions.
- Rain and Snow: Reduced visibility due to precipitation can hinder the sensors’ ability to accurately perceive the surroundings. This can lead to misjudgments in distance, speed, and object identification. Systems may also struggle with maintaining traction on wet or icy surfaces.
- Fog and Low Visibility: Significant reductions in visibility due to fog can significantly affect sensor performance. This makes it harder for the system to perceive obstacles and react in a timely manner. The sensors might have difficulty distinguishing between objects and the surrounding environment.
- Strong Winds: Strong winds can affect the stability of both the self-driving vehicle and other vehicles on the road. This poses a challenge for the system in maintaining control and predicting the behavior of other vehicles. The unpredictability of wind gusts and their impact on surrounding vehicles can result in collisions.
Role of Road Infrastructure
Road infrastructure significantly influences the safety of self-driving vehicles. Poorly maintained roads, poorly marked lanes, and inconsistent signage can contribute to accidents. This is especially pertinent in areas where the infrastructure is not optimized for autonomous systems.
- Poor Road Maintenance: Potholes, uneven surfaces, and damaged road markings can negatively affect the performance of the self-driving vehicle’s sensors and its ability to navigate safely. Such imperfections might confuse the system’s perception of the road layout and lead to unexpected maneuvers.
- Inadequate Signage and Lane Markings: Inconsistent or poorly maintained lane markings, signs, and traffic signals can confuse the self-driving vehicle’s navigation system. This may result in improper lane changes or incorrect interpretations of traffic flow.
- Complex Intersections: Intersections with multiple traffic patterns and poorly designed layouts can be challenging for self-driving systems. The system’s ability to accurately interpret traffic signals, predict the movements of other vehicles, and safely navigate through the intersection is paramount.
Impact of Human Error
While the focus is on the autonomous system, human error can still play a role in self-driving car accidents. This includes factors like interactions with other vehicles or pedestrians. In scenarios where the self-driving vehicle is responding to human actions or interactions, human error can contribute to an accident.
- Driver Interaction: In situations where the human driver is expected to intervene, a lack of clarity in responsibilities or an improper response from the human driver can lead to an accident.
- Other Vehicle Actions: Actions from other vehicles, like sudden lane changes or improper stops, can affect the self-driving vehicle’s response time. The ability of the autonomous system to predict and react to these unpredictable actions is crucial.
- Pedestrian Actions: As previously noted, the unpredictability of pedestrian behavior can lead to accidents. The system’s ability to anticipate and react to unexpected actions by pedestrians is crucial.
Comparison with Traditional Accidents
A crucial aspect of evaluating self-driving car technology is comparing its accident rate and types to those of conventional, human-driven vehicles. Understanding the differences can illuminate areas needing improvement and highlight the unique challenges associated with autonomous systems. This comparison also helps to contextualize the safety benefits and risks associated with the transition to self-driving cars.The existing data on self-driving car accidents, while still limited, suggests a different accident profile compared to traditional accidents.
While self-driving cars are designed to mitigate human error, new types of failures and vulnerabilities may emerge, requiring specific preventative strategies.
Frequency of Accidents
The frequency of accidents involving self-driving cars is significantly lower than that of human-driven vehicles, according to the available data. This initial advantage, however, may change as the technology matures and encounters more complex driving scenarios. The current trend indicates fewer accidents, but this must be viewed within the context of limited deployment and relatively low operational hours for self-driving vehicles.
Accident Types
The types of accidents involving self-driving cars differ from those of human-driven cars. Self-driving cars tend to be involved in accidents stemming from interactions with other vehicles, pedestrian mishaps, and sometimes, unexpected environmental factors. Human-driven accidents frequently involve driver error, distracted driving, and issues related to human judgment and reaction time. The breakdown in the types of accidents highlights specific areas where self-driving systems need refinement.
Self-driving car accident statistics are still a concern, and researchers are actively looking for ways to improve safety. One promising avenue involves using generative AI tools to simulate various driving scenarios and identify potential hazards. These simulations could help in creating more robust safety protocols and predictive models for self-driving car development, ultimately leading to fewer accidents. Understanding the potential of Generative AI tools in this context is crucial for future advancements in autonomous vehicle technology.
Contributing Factors
The contributing factors to accidents vary between human-driven and self-driving vehicles. For human-driven cars, the primary factors often include driver fatigue, inattention, or poor judgment. In self-driving car accidents, factors like sensor malfunction, inadequate mapping data, or flawed algorithms are prominent concerns.
Accident Prevention Strategies
Accident prevention strategies differ markedly between the two categories. For human-driven cars, strategies focus on driver education, stricter enforcement of traffic laws, and the promotion of safe driving practices. Self-driving car safety measures emphasize the development of robust sensor systems, improved algorithms, more comprehensive and accurate maps, and enhanced vehicle safety features to mitigate potential failures.
Key Differences in Accident Patterns
Category | Human-Driven Accidents | Self-Driving Accidents |
---|---|---|
Primary Contributing Factor | Driver error (e.g., distracted driving, speeding, fatigue) | System malfunction (e.g., sensor failure, algorithmic errors, inadequate mapping) |
Accident Type | Rear-end collisions, lane departures, collisions with pedestrians or cyclists | Collisions with other vehicles, pedestrian mishaps, environmental hazards (e.g., sudden obstacles) |
Prevention Strategy | Driver training, improved traffic infrastructure, promoting safe driving habits | Advanced sensor technology, improved algorithms, enhanced vehicle safety systems |
Technological Failures and Accidents
Self-driving cars, while promising a future of safer transportation, are not immune to technological glitches. Failures in the underlying software, sensors, and algorithms can lead to unexpected and potentially dangerous situations. Understanding these vulnerabilities is crucial for improving safety protocols and enhancing the reliability of autonomous vehicles.
Potential Technological Failures
Various technological malfunctions can contribute to accidents involving self-driving cars. These failures can range from minor software glitches to more serious hardware malfunctions, each with varying degrees of impact. Factors like the vehicle’s environment, the complexity of the software, and the sophistication of the sensor technology play a significant role in the potential for such failures.
Software Bugs and Algorithm Errors
Software bugs and algorithm errors are a significant concern. Faulty programming can lead to misinterpretations of the environment, incorrect decision-making by the vehicle’s control systems, and ultimately, accidents. For example, if an algorithm fails to recognize a pedestrian in a specific lighting condition or a poorly marked crosswalk, the vehicle may not react appropriately, potentially resulting in a collision.
Furthermore, unexpected inputs, such as extreme weather conditions, can also trigger malfunctions in the algorithms. The sophistication and complexity of the algorithms used in self-driving vehicles make them susceptible to unforeseen errors.
Sensor and Camera Malfunctions
Sensors and cameras are the eyes and ears of the self-driving car, providing real-time data about the environment. Malfunctions in these crucial components can lead to inaccurate or incomplete data, which can result in the vehicle misinterpreting its surroundings. For example, a malfunctioning camera might fail to capture a critical detail, such as a cyclist hidden by shadows, potentially causing a collision.
Furthermore, sensor failures, like those due to interference from other electronic devices, can lead to incorrect readings of distances and speeds. The reliability and accuracy of sensor data are paramount to the safe operation of self-driving cars.
Impact of Technological Malfunctions
Type of Malfunction | Impact on Safety | Example |
---|---|---|
Software Bug (Object Recognition) | Vehicle may misinterpret objects, leading to collisions. | A pedestrian obscured by sunlight is not detected. |
Sensor Malfunction (Distance Measurement) | Vehicle may misjudge distance to obstacles, resulting in collisions. | A sensor measures a shorter distance to a parked car than it actually is. |
Camera Malfunction (Image Processing) | Vehicle may fail to identify important objects in the environment, leading to collisions. | A camera fails to capture a cyclist hidden by a large truck. |
Algorithm Error (Traffic Signal Interpretation) | Vehicle may misinterpret traffic signals, leading to accidents. | A vehicle misinterprets a yellow light as a green light. |
This table highlights the various types of technological malfunctions and their potential impact on safety. Addressing these vulnerabilities is essential for the development of reliable and safe self-driving vehicles.
Public Perception and Safety Concerns
Public perception of self-driving car safety is a crucial factor in the technology’s adoption and societal acceptance. Concerns about the technology’s reliability and the potential for accidents are significant hurdles that need to be addressed to foster public trust. The public’s perception is often shaped by media coverage, personal experiences, and the perceived risks and benefits.Public perception of self-driving car safety is multifaceted, involving not only the technology’s safety record but also societal anxieties about its impact on various aspects of life.
This intricate interplay between technological advancement and public opinion plays a pivotal role in the widespread adoption of self-driving vehicles.
Public Perception of Self-Driving Car Safety
Public perception of self-driving car safety is influenced by various factors, including the frequency and severity of reported accidents, the perceived competence of the technology in handling unforeseen situations, and the perceived reliability of the safety systems. The public’s understanding of the technology’s limitations and capabilities is also a key element.
Concerns and Fears about Self-Driving Car Accidents
Concerns about self-driving car accidents are often tied to a lack of understanding about how these systems operate. Fears range from concerns about the technology’s ability to react to unpredictable situations to worries about liability in case of accidents. The public also often grapples with the idea of relying on a machine for their safety, raising questions about human control and responsibility.
Statistics Regarding Public Confidence in Self-Driving Cars, Self-driving car accidents statistics
Public confidence in self-driving cars varies significantly depending on the specific context and the individuals surveyed. Surveys and studies consistently show that public confidence in the technology’s ability to prevent accidents is not uniform across demographics and geographical regions. Furthermore, levels of confidence often correlate with the individual’s level of familiarity with the technology and their personal experiences with its use.
Public’s Perception of the Technology’s Reliability
The public’s perception of self-driving car reliability is a complex issue. While the technology shows potential, there are still uncertainties about its long-term performance and ability to adapt to various environmental conditions. Public perception of reliability is also influenced by media reports of accidents, incidents, and malfunctions. Moreover, the lack of widespread deployment and real-world experience further impacts the public’s perception of reliability.
This uncertainty often leads to hesitation about adopting this technology.
Accident Investigation and Reporting Procedures
Accident investigation procedures for self-driving cars are crucial for understanding the root causes of incidents, implementing preventative measures, and enhancing public trust. These processes must be transparent, rigorous, and standardized to ensure accountability and effective learning from past events. Robust reporting mechanisms are essential to track accident trends and identify potential areas for improvement in the technology and operational procedures of self-driving vehicles.
Investigation Process Overview
The investigation process for self-driving car accidents typically involves a multi-faceted approach, encompassing technical analysis of the vehicle’s systems, examination of environmental factors, and review of human interactions. Investigators employ a systematic methodology to gather data from various sources, such as sensor data from the vehicle, eyewitness accounts, and traffic data. The meticulous collection and analysis of this data are essential for identifying the cause of the accident.
Stakeholder Roles in Investigation
The investigation process necessitates the involvement of various stakeholders. Automakers play a key role in examining the vehicle’s software, hardware, and sensor performance. Insurance companies assess the financial implications and liabilities related to the accident. Government agencies are responsible for ensuring compliance with safety regulations and overseeing the investigation process. Independent experts, such as engineers and accident reconstruction specialists, provide crucial technical insights and analyses.
Law enforcement agencies are often involved in the investigation to establish liability and gather evidence.
Accident Reporting Procedures
Detailed reporting procedures are necessary to document accident data comprehensively. This includes the specific details of the accident, such as location, time, weather conditions, and contributing factors. The reporting process should also include data on the self-driving system’s behavior during the incident, including sensor readings, system logs, and any potential malfunctions. Data must be organized in a standardized format to facilitate analysis and comparison across different incidents.
Reporting procedures must adhere to established guidelines to ensure transparency and facilitate effective communication between stakeholders.
Preventive Measures and Future Improvements
The investigation of self-driving car accidents can lead to significant improvements in safety. Identifying recurring patterns and causes allows for targeted development of preventative measures. This includes refining algorithms, enhancing sensor technology, improving system redundancy, and implementing more comprehensive safety protocols. For example, if a particular type of sensor malfunction is consistently linked to accidents, engineers can implement measures to mitigate the risk of such malfunctions.
The insights gained from investigations can lead to more robust and resilient self-driving systems, ultimately reducing the frequency and severity of accidents.
Regulatory Frameworks and Safety Standards
Current regulations for self-driving cars are evolving rapidly, aiming to strike a balance between innovation and safety. The absence of comprehensive, globally harmonized standards poses a challenge, leading to inconsistencies in safety requirements and potentially impacting the widespread adoption of this technology. This necessitates the development of specific safety standards tailored to the unique characteristics of autonomous vehicles.The current regulatory landscape for self-driving cars is fragmented, with different countries and regions implementing various approaches.
This lack of uniformity creates uncertainty for manufacturers, potentially hindering innovation and global market penetration. The urgent need for harmonized standards will be vital for fostering public trust and confidence in this emerging technology.
Existing Regulatory Frameworks
Current regulations often treat self-driving cars as extensions of traditional vehicles, applying existing traffic laws and safety standards. However, this approach may not fully address the unique safety concerns associated with autonomous systems. The complexity of algorithms, sensor technologies, and decision-making processes in self-driving vehicles necessitates specific considerations.
Need for Specific Safety Standards
The complexity of self-driving vehicle systems demands tailored safety standards. These standards should encompass not only the vehicle’s hardware and software but also the operational procedures and testing protocols for autonomous systems. Addressing potential vulnerabilities in algorithms, sensor limitations, and cybersecurity threats is critical for safety. Standards should Artikel requirements for data logging, incident reporting, and continuous system improvement.
Comparison of Regulations Across Countries
Regulatory frameworks for self-driving cars vary significantly across countries. Some jurisdictions have adopted a more cautious approach, emphasizing safety and stringent testing requirements, while others have been more lenient, potentially leading to a divergence in safety standards and market penetration. For instance, the United States has a patchwork of state-level regulations, contrasting with the more centralized approach of countries like Germany.
Summary of Key Regulations and Standards
Country | Key Regulations/Standards | Focus Areas |
---|---|---|
United States | State-level regulations, varying significantly. Some states have specific legislation for autonomous vehicles. | Safety testing, vehicle design, data privacy. |
Europe | Harmonization efforts through the EU, but national variations persist. | Safety standards, cybersecurity, liability issues. |
China | National regulations, often focused on innovation while prioritizing safety. | Integration with existing infrastructure, development of testing facilities. |
Japan | Specific regulations for autonomous vehicle testing and deployment. | Infrastructure adaptation, public acceptance. |
“A lack of clear and consistent regulations poses a significant challenge for the widespread adoption of self-driving technology.”
Future Trends and Predictions
The ongoing development of self-driving car technology presents both exciting opportunities and complex challenges regarding safety. Understanding future trends in accident statistics is crucial for refining safety protocols and public perception. This section explores anticipated improvements in safety technology, projections for accident rates, and the impact of emerging technologies.
Anticipated Improvements in Self-Driving Car Safety Technology
Advancements in sensor technology, machine learning algorithms, and communication protocols are expected to significantly enhance the safety of self-driving vehicles. Improved sensor fusion, enabling vehicles to better interpret and respond to complex scenarios, is a key focus. For example, lidar and radar systems are being refined to provide more accurate and comprehensive data, enabling the car to perceive obstacles and surroundings with greater precision.
Simultaneously, AI-driven algorithms are being trained on vast datasets of real-world driving scenarios, allowing them to learn and adapt to various situations more effectively. This includes better handling of unexpected situations and improving reaction times. Enhanced communication capabilities between vehicles and infrastructure will allow for better coordination and decision-making in traffic, thus reducing the risk of accidents.
Projected Future Trends in Self-Driving Car Accident Statistics
Analyzing historical data and projecting future trends in self-driving car accident statistics requires careful consideration of evolving technologies and their impact. The expectation is that accident rates will decline as safety features are refined and implemented. However, the specific rate of reduction will depend on various factors, including the rate of adoption of self-driving technology, the effectiveness of safety regulations, and the extent of human oversight in critical situations.
The initial years of deployment will likely witness a higher number of accidents, as self-driving systems learn and adapt to new environments and driving conditions. However, as these systems mature and become more refined, the accident rate is anticipated to decrease, ultimately approaching or even surpassing the accident rate of human-driven vehicles. The ultimate goal is a significant reduction in accidents, leading to safer roads for everyone.
Potential Impact of Emerging Technologies on Accident Rates
Emerging technologies, such as 5G communication networks and advanced driver-assistance systems (ADAS), have the potential to significantly influence accident rates in self-driving vehicles. 5G networks, with their enhanced bandwidth and low latency, can facilitate faster communication between vehicles, improving coordination and responsiveness during critical moments. This allows for more efficient sharing of information among vehicles, reducing the possibility of collisions.
ADAS features, like advanced braking systems and lane-keeping assistance, can complement self-driving systems, enhancing safety in various situations. The integration of these technologies with self-driving capabilities is anticipated to lead to a substantial decrease in accident rates compared to traditional driving.
Prediction of Accident Rates Evolution in the Next 5-10 Years
Predicting accident rates over the next 5-10 years is complex and depends on several variables. While a significant reduction is anticipated, the exact rate of decrease will be influenced by the pace of technological advancements, regulatory frameworks, and public acceptance of self-driving vehicles. Some industry experts predict a gradual decline in accident rates, potentially reaching a level comparable to or even lower than that of traditional vehicles within the next decade.
However, it is essential to recognize that unforeseen challenges and emerging issues could impact these predictions. Moreover, the effectiveness of safety measures will depend on factors such as the diversity of driving conditions, the availability of reliable data, and the implementation of appropriate safety protocols.
Ultimate Conclusion
In conclusion, while self-driving car accidents statistics show progress in safety, there are still significant challenges to overcome. Addressing technological failures, improving public perception, and implementing robust regulatory frameworks are crucial steps towards ensuring the safe and widespread adoption of autonomous vehicles. Further research and data collection will be essential for refining safety protocols and improving future designs.
FAQ
What are the most common types of self-driving car accidents?
Common accident types include collisions with pedestrians, cyclists, and other vehicles. Weather conditions and road infrastructure also play a role.
How do self-driving car accident rates compare to human-driven car accidents?
This is a complex comparison, and rates vary depending on the specific metrics used. Data is still emerging and requires careful analysis to draw definitive conclusions.
What role do technological malfunctions play in self-driving car accidents?
Software bugs, algorithm errors, and sensor/camera malfunctions can contribute to accidents. The specific impact of each type of malfunction is being investigated and documented.
What are the key safety concerns regarding self-driving cars?
Public perception of safety, reliability, and the ethical implications of accidents are significant concerns. The lack of clear legal frameworks for these accidents also presents challenges.