Car crash detection and prevention is a crucial area of research and development, aiming to minimize road accidents. This field encompasses a wide range of technologies, from advanced sensor systems to sophisticated data analysis techniques, all working together to improve road safety.
The evolution of car crash detection and prevention has been marked by significant advancements. Early systems focused primarily on reactive measures, but modern approaches prioritize proactive strategies. This includes everything from driver assistance systems to infrastructure improvements, all designed to prevent crashes before they occur. Different types of crashes, such as frontal, rear-end, and side impacts, each present unique challenges that must be addressed to achieve the best results.
Introduction to Car Crash Detection and Prevention
The field of car crash detection and prevention is a critical area of research and development, aiming to reduce the devastating impact of traffic accidents. This endeavor has evolved significantly over the years, moving from basic accident analysis to sophisticated systems that proactively mitigate potential collisions. Understanding the types of crashes, their contributing factors, and the fundamental principles behind detection and prevention is crucial for improving road safety globally.The significance of this field is undeniable.
Millions of people are injured or killed annually in car crashes worldwide, leading to substantial economic burdens and profound human suffering. Innovative approaches to crash detection and prevention hold the key to reducing these tragic outcomes and building safer transportation systems.
Types of Car Crashes and Contributing Factors
Car crashes manifest in various forms, each with unique contributing factors. Understanding these diverse crash types is fundamental to developing targeted prevention strategies. This section details common crash types and their associated causes.
- Frontal crashes, often the most severe, frequently result from driver error, such as speeding, distracted driving, or failing to maintain a safe following distance. Environmental factors, such as poor weather conditions or road hazards, can also significantly increase the risk of frontal collisions.
- Rear-end collisions commonly occur due to inadequate following distance, a prevalent issue among drivers who are inattentive or not responding quickly to traffic conditions. Sudden braking by the vehicle in front or the driver’s reaction time are often critical factors.
- Side-impact crashes can arise from various sources, including lane departures, turning errors, or the actions of other road users. These collisions can be particularly dangerous due to the potential for injuries to occupants on the impacted side of the vehicle.
- Rollover crashes often occur when a vehicle exceeds the limits of its stability during sharp turns, driving on uneven surfaces, or experiencing high speeds. These accidents are frequently linked to factors like poor vehicle design or driving habits that exacerbate the likelihood of losing control.
Fundamental Principles of Crash Detection and Prevention
The fundamental principles of car crash detection and prevention rely on identifying potential hazards and mitigating their impact. This involves proactive measures that anticipate and respond to dangerous situations before they escalate into accidents.
- Advanced driver-assistance systems (ADAS) play a critical role by providing drivers with real-time information about potential dangers, such as forward collision warnings or lane departure alerts. These systems provide alerts, giving the driver a chance to react and mitigate the potential for a crash.
- Improved vehicle design incorporates safety features, such as reinforced structures and enhanced braking systems, that can minimize the severity of impacts in the event of a collision. These features aim to lessen the harm to the vehicle’s occupants.
- Infrastructure improvements, such as better road markings, improved signage, and safer intersections, reduce the likelihood of accidents by providing clear guidance and warnings to drivers. Improved infrastructure, alongside technological improvements, is key to enhanced road safety.
Successful Crash Prevention Strategies
Several successful crash prevention strategies have been implemented in various contexts, demonstrating the efficacy of proactive approaches.
- Many cities have implemented speed limits and enforcement campaigns, which have proven effective in reducing the frequency of crashes involving speeding.
- Public awareness campaigns and driver education programs that emphasize safe driving practices, such as maintaining safe following distances and avoiding distractions, have been proven successful in altering driver behavior and reducing the rate of collisions.
- Automated emergency braking systems (AEBS) are integrated into many new vehicles, significantly reducing rear-end collisions and front-end crashes, as the system detects and initiates braking actions in hazardous situations. This demonstrates how technology is being utilized to mitigate accident risk.
Car Crash Categories and Common Causes
This table Artikels different car crash categories and their common causes.
Crash Category | Common Causes |
---|---|
Frontal | Speeding, distracted driving, following too closely, adverse weather conditions, road hazards |
Rear-end | Following too closely, inattentive driving, sudden braking, reduced visibility |
Side Impact | Lane departures, turning errors, actions of other road users, impaired visibility |
Rollover | High speeds, sharp turns, uneven surfaces, poor vehicle design, driving habits |
Sensor Technologies for Crash Detection
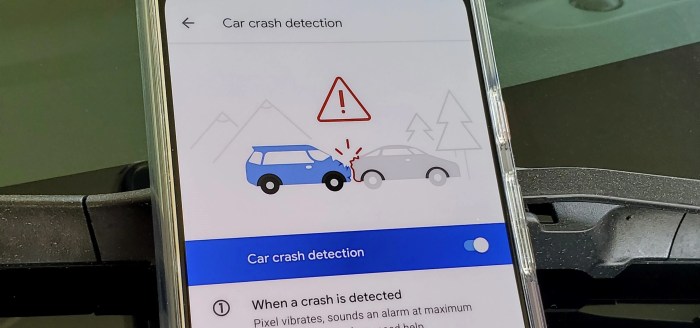
A critical component of modern crash detection and prevention systems is the deployment of various sensor technologies. These sensors play a pivotal role in monitoring vehicle dynamics, environmental conditions, and driver behavior, enabling early detection of potential hazards. Accurate and reliable sensing is essential for triggering appropriate safety interventions, ultimately minimizing the risk of accidents.
Different Types of Sensors
Various sensor types contribute to the comprehensive picture of potential crashes. These range from readily available technologies to more specialized systems. Each sensor type possesses unique characteristics that influence its effectiveness in crash detection.
- Cameras: Video cameras, often multiple and strategically positioned, are widely used. They capture visual data, enabling real-time monitoring of the surrounding environment, including traffic conditions, road markings, and other vehicles. The camera’s field of view, resolution, and frame rate significantly impact its ability to detect potential hazards. Furthermore, image processing algorithms are used to identify objects, estimate distances, and detect anomalies in traffic flow.
- Radar Sensors: Radar systems, employing radio waves, provide accurate distance measurements and velocity data. They can detect obstacles and track their movement, enabling proactive responses to potential collisions. Radar’s ability to operate in various weather conditions and its high accuracy make it a valuable component in crash prevention systems.
- Lidar Sensors: Light Detection and Ranging (LiDAR) systems utilize laser pulses to create detailed 3D maps of the surroundings. LiDAR provides high-resolution data, enabling precise distance measurements and object identification. This technology is particularly useful in complex environments with multiple obstacles, providing a more comprehensive picture of the driving environment.
- Inertial Measurement Units (IMUs): IMUs measure acceleration, angular velocity, and orientation of the vehicle. This data is crucial in detecting sudden changes in motion, which could indicate a potential collision. These sensors, often integrated into advanced driver-assistance systems (ADAS), are effective in recognizing sudden maneuvers and alerting the driver or initiating autonomous braking.
- GPS Sensors: GPS sensors provide information about the vehicle’s location and speed. This data, combined with other sensor inputs, helps to track the vehicle’s trajectory and identify potential deviations from the expected path, which could signal a collision risk.
Principles of Operation
The operating principles of each sensor type differ significantly. Understanding these principles provides insight into the strengths and limitations of each technology.
- Cameras: Cameras capture images of the environment, which are then processed by algorithms to identify objects and track their movements. Object recognition and distance estimation are critical aspects of this process. Sophisticated algorithms and image processing techniques improve accuracy.
- Radar Sensors: Radar sensors emit radio waves, and the time it takes for these waves to reflect back from an object is used to determine the distance and velocity of the object. This technology is robust in various weather conditions. Sophisticated radar systems use multiple beams to generate a detailed view of the surroundings.
- Lidar Sensors: LiDAR sensors emit laser pulses, and the time taken for the reflected light to return determines the distance to an object. The strength and pattern of the reflected light provide additional information about the object. This enables detailed 3D mapping of the environment.
- IMUs: IMUs measure acceleration and angular velocity using accelerometers and gyroscopes. Sudden changes in these measurements indicate potential collisions. The data is continuously monitored and compared with expected vehicle behavior.
- GPS Sensors: GPS sensors receive signals from satellites to determine the vehicle’s location and speed. Combined with other sensor data, GPS can identify deviations from the expected path, which might indicate a potential hazard.
Sensor Comparison
A comparative analysis of sensor technologies is crucial for selecting the optimal sensors for a specific crash detection system. Each sensor has strengths and weaknesses in terms of accuracy, cost, and reliability.
Sensor Type | Accuracy | Cost | Reliability |
---|---|---|---|
Cameras | Moderate to High | Low to Moderate | Moderate to High |
Radar | High | Moderate to High | High |
LiDAR | High | High | High |
IMUs | High | Low to Moderate | High |
GPS | Moderate | Low | Moderate |
Sensor Applications
Real-world applications of these sensor technologies are widespread. Integration into various systems, such as ADAS and autonomous driving, is increasing.
- Autonomous Emergency Braking (AEB): Radar and camera systems are frequently used in AEB systems to detect vehicles or pedestrians in front of the car and initiate braking if a collision is imminent.
- Lane Departure Warning Systems (LDWS): Cameras and IMUs can detect when a vehicle is drifting out of its lane, alerting the driver to the potential hazard.
- Blind Spot Monitoring Systems (BSM): Radar sensors detect vehicles in the driver’s blind spots, alerting the driver of potential collisions.
Data Processing and Analysis for Crash Detection: Car Crash Detection And Prevention
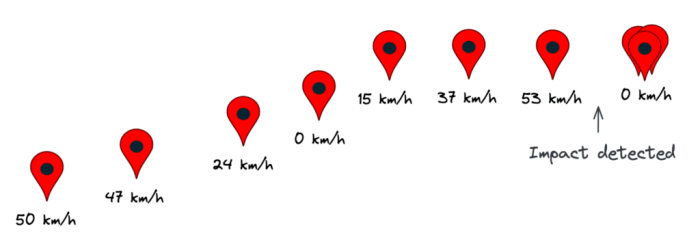
Effective car crash detection hinges on the meticulous processing and analysis of sensor data. This stage transforms raw sensor inputs into actionable insights, enabling the system to accurately identify potential crash scenarios. The crucial steps involved in this process ensure the system’s reliability and efficiency.
Data Collection and Preprocessing
Raw sensor data, collected from various sources, often needs significant preparation before analysis. This initial step, data preprocessing, involves cleaning, transforming, and organizing the data to enhance its usability for algorithms. Crucially, this stage addresses inconsistencies, outliers, and missing values that could otherwise negatively impact the accuracy of crash detection.
Data Cleaning Techniques
Various techniques are employed to cleanse the data. Imputation methods fill in missing data points, while outlier detection and removal techniques identify and eliminate values that deviate significantly from the expected range. Data transformation methods, such as normalization or standardization, ensure data consistency and prevent certain features from dominating the analysis. These steps collectively contribute to a more reliable dataset.
Data Transformation Methods
Normalizing or standardizing the data ensures that different features don’t unduly influence the analysis. For example, sensor readings with different scales (e.g., GPS coordinates vs. acceleration values) can be normalized to a common range. This prevents features with larger numerical values from disproportionately affecting the model’s performance. Feature scaling techniques are crucial to prevent bias.
Crash Situation Identification Algorithms
Sophisticated algorithms analyze the preprocessed data to identify potential crash situations. Machine learning algorithms, such as support vector machines (SVMs), decision trees, and neural networks, play a pivotal role in this stage. These algorithms learn patterns and relationships within the data, enabling them to predict potential crashes based on the gathered sensor readings.
Data Preprocessing and Cleaning Importance
Data preprocessing and cleaning are paramount. Inaccurate or incomplete data can lead to flawed crash detection predictions, resulting in false positives or negatives. By ensuring the data is accurate and consistent, the reliability of the detection system is significantly improved. Furthermore, a clean dataset is essential for the effective training of machine learning models.
Machine Learning Models for Crash Detection
Various machine learning models are employed in crash detection systems. Support Vector Machines (SVMs) excel at classification tasks, effectively separating crash scenarios from normal driving situations. Decision trees provide a clear and interpretable structure for identifying the contributing factors to a potential crash. Neural networks, particularly deep learning models, are capable of capturing complex patterns and relationships in sensor data, potentially leading to enhanced accuracy.
Each model offers a unique approach, and the selection often depends on the specific requirements and characteristics of the dataset.
Data Processing Stages, Car crash detection and prevention
Stage | Description |
---|---|
Data Collection | Gathering sensor data from various sources (e.g., accelerometers, GPS, cameras). |
Data Preprocessing | Cleaning, transforming, and organizing the collected data to prepare it for analysis. |
Feature Engineering | Extracting relevant features from the data, often using mathematical or statistical transformations, to represent the essential characteristics of the situation. |
Model Training | Training machine learning models using the preprocessed data to identify crash patterns. |
Model Evaluation | Evaluating the model’s performance using metrics such as precision, recall, and F1-score. |
Driver Assistance Systems (ADAS) and Crash Prevention
Driver assistance systems (ADAS) are increasingly crucial in mitigating traffic accidents. They utilize various technologies to enhance driver awareness and control, thereby reducing the likelihood of collisions. ADAS systems offer a range of functionalities, from simple warnings to fully automated interventions, contributing significantly to safer driving practices.
Role of ADAS in Mitigating Crashes
ADAS systems play a vital role in preventing crashes by actively assisting drivers in hazardous situations. These systems enhance driver awareness and reaction time, enabling them to make informed decisions and react more effectively to potential hazards. Through advanced sensors and sophisticated algorithms, ADAS systems can detect potential dangers before they become accidents.
Functionalities of ADAS Features
Various ADAS features contribute to improved safety. These features include lane departure warnings, adaptive cruise control, and automatic emergency braking. Understanding their individual functionalities is essential to appreciate their collective impact on crash prevention.
- Lane Departure Warning (LDW): LDW systems use sensors, typically cameras, to monitor the vehicle’s position within the lane markings. When the vehicle drifts out of its lane without a turn signal activated, the system alerts the driver with visual and/or auditory signals. This proactive intervention can help prevent accidents caused by unintended lane departures, especially in drowsy driving or distracted driving scenarios.
- Adaptive Cruise Control (ACC): ACC systems maintain a preset distance from the vehicle ahead. When the vehicle in front slows down, the ACC system automatically adjusts the speed to maintain the pre-set distance. This helps avoid rear-end collisions and contributes to more consistent and safer following distances.
- Automatic Emergency Braking (AEB): AEB systems use sensors to detect vehicles or pedestrians in the path of the vehicle. If a collision is imminent, the system automatically applies the brakes to mitigate the severity of the collision or, in some cases, to prevent the collision entirely. AEB systems vary in their sophistication, with some systems capable of detecting and responding to both vehicles and pedestrians.
Advantages and Disadvantages of Different ADAS Systems
ADAS systems offer substantial advantages in terms of safety, but they also have certain limitations.
- Advantages: Improved driver safety, reduced risk of accidents, increased awareness of surroundings, and potentially reduced insurance premiums are among the key advantages. Systems such as ACC can reduce driver fatigue and stress by managing speed control, while AEB systems can significantly reduce accident severity.
- Disadvantages: ADAS systems can be expensive, and their effectiveness can be influenced by environmental factors like weather conditions or poor visibility. There are also potential issues with false alarms, where the system might trigger a warning or intervention when no danger is present. Furthermore, over-reliance on ADAS systems might lead to a decrease in driver attentiveness.
Comparison and Contrast of ADAS Features
Comparing the effectiveness of different ADAS features reveals distinct strengths and weaknesses. For instance, while lane departure warnings can be highly effective in preventing unintended lane departures, their effectiveness is limited in complex or poorly marked driving environments. Adaptive cruise control, on the other hand, is particularly helpful in maintaining a safe following distance, but its performance is influenced by the accuracy and responsiveness of the sensors.
AEB systems demonstrate high potential for preventing or mitigating collisions, but their accuracy depends on various factors, including sensor reliability and the specific circumstances of the collision.
Summary Table of ADAS Functionalities and Effectiveness
ADAS Feature | Functionality | Effectiveness | Limitations |
---|---|---|---|
Lane Departure Warning | Alerts driver of unintended lane departure | High, especially in predictable environments | Limited in complex or poorly marked lanes |
Adaptive Cruise Control | Maintains safe following distance | High, but influenced by sensor accuracy | Can be affected by environmental conditions |
Automatic Emergency Braking | Automatically applies brakes in emergency situations | High potential, but subject to accuracy of sensors | Can trigger false alarms |
Vehicle Infrastructure Integration for Crash Prevention
Integrating vehicles with intelligent infrastructure is a crucial step towards enhancing road safety and reducing accidents. This integration leverages real-time data exchange and advanced sensor technologies to provide drivers with crucial information and support, thereby enabling proactive responses to potential hazards. Smart systems can anticipate potential collisions and alert drivers, reducing reaction time and mitigating the severity of accidents.The integration of vehicles with infrastructure, encompassing traffic signals, road markings, and other infrastructure components, plays a critical role in proactively detecting and preventing accidents.
Advanced sensor technologies within vehicles and infrastructure allow for continuous monitoring of traffic flow, environmental conditions, and potential hazards. This continuous monitoring, coupled with data sharing, creates a network of interconnected systems that work together to enhance safety.
Smart Traffic Signals and Road Infrastructure
Smart traffic signals, equipped with sensors and advanced algorithms, can dynamically adjust signal timings based on real-time traffic conditions. This optimized traffic flow minimizes congestion, reduces stop-and-go driving, and thus decreases the likelihood of accidents caused by sudden braking and acceleration. Similarly, intelligent road infrastructure can incorporate features like embedded sensors that detect potential hazards, such as potholes or debris, providing timely warnings to drivers.
Car crash detection and prevention systems are becoming increasingly sophisticated. This advancement relies heavily on robust software and algorithms, often developed by companies specializing in Software & App Development. These sophisticated systems are ultimately crucial for improving road safety and reducing accidents.
This proactive approach can help prevent accidents.
Data Sharing Between Vehicles and Infrastructure
Data sharing between vehicles and infrastructure is paramount for enhanced crash detection. Vehicles equipped with advanced sensors can transmit data on their location, speed, and direction to the infrastructure. This information, combined with traffic flow data from sensors in the road, allows for the creation of a comprehensive picture of the road environment. This holistic view of traffic conditions enables the infrastructure to provide crucial alerts to drivers, such as potential hazards ahead or changes in traffic flow.
For instance, a vehicle approaching a construction zone could receive an alert from the infrastructure, potentially preventing an accident.
Real-Time Traffic Data for Preemptive Measures
Real-time traffic data is essential for preemptive measures to prevent accidents. This data, collected from various sources, including sensors in the road and vehicles, allows for the prediction of potential traffic congestion or hazards. Using this data, traffic management systems can adjust signal timings, reroute traffic, or provide warnings to drivers in advance, thereby mitigating the risk of accidents.
Predictive modeling based on historical and real-time data can help identify high-risk areas and proactively address potential problems, reducing the likelihood of incidents.
Types of Infrastructure-Vehicle Integration and Benefits
Type of Integration | Description | Benefits |
---|---|---|
Connected Vehicle Infrastructure | Vehicles and infrastructure communicate directly using various communication protocols, enabling real-time data exchange. | Enhanced safety by providing real-time information to drivers, improved traffic flow through dynamic signal adjustments, and reduced congestion. |
Smart Roadway Sensors | Sensors embedded in the roadway detect obstacles, hazards, and traffic conditions, transmitting this data to vehicles and traffic management systems. | Early detection of potential hazards like potholes or debris, improved traffic management, and increased safety for drivers. |
Dynamic Traffic Signal Systems | Signal timings are adjusted based on real-time traffic conditions, optimizing traffic flow and minimizing delays. | Reduced congestion, minimized stop-and-go driving, and reduced accident risk. |
Real-world examples of infrastructure-vehicle integration include pilot projects in various cities around the world, demonstrating the potential for safer and more efficient traffic management.
Human Factors and Crash Prevention
Human factors play a critical role in the majority of car crashes, often exceeding the influence of mechanical failures or environmental conditions. Understanding these factors is crucial for developing effective strategies to reduce accidents. This involves recognizing the multifaceted nature of driver behavior and how it interacts with vehicle systems and infrastructure.Driver behavior is a complex interplay of individual characteristics, environmental pressures, and the vehicle’s design.
Factors like attention, perception, and decision-making directly influence the driver’s ability to react appropriately to situations on the road. Addressing these human elements alongside technological advancements is essential for comprehensive crash prevention strategies.
Impact of Driver Distraction
Driver distraction, whether from cell phones, in-car entertainment systems, or conversations, significantly reduces a driver’s situational awareness. This diminished awareness can lead to delayed responses to hazards, incorrect lane changes, or inadequate braking, all increasing the risk of accidents. Distraction often compromises the driver’s ability to effectively process visual and auditory information, making them less responsive to potential dangers.
For example, studies have shown that using a cell phone while driving significantly increases the risk of an accident.
Impact of Driver Fatigue
Driver fatigue is another critical human factor contributing to crashes. Extended driving periods, inadequate rest, and sleep deprivation can impair cognitive functions, reaction times, and decision-making. These impairments can manifest as drowsiness, reduced alertness, and a decreased ability to perceive and react to changing road conditions. The effects of fatigue are similar to those of alcohol impairment, highlighting the importance of rest breaks and adherence to driving regulations regarding fatigue management.
Impact of Driver Inattentiveness
Inattentiveness encompasses a broader range of driver behaviors, including daydreaming, inattention to traffic signs, and a lack of focus on the road ahead. Inattention can be attributed to factors such as boredom, stress, or even poor road design. Inattentive driving can result in misjudged distances, incorrect lane changes, and inadequate responses to sudden changes in traffic flow, all of which can contribute to collisions.
Role of Driver Training and Education
Driver training and education programs play a vital role in minimizing the impact of human factors. Effective programs focus on enhancing driver skills, increasing awareness of hazards, and improving decision-making capabilities. These programs typically include theoretical knowledge and practical exercises to develop better reaction times, safe driving habits, and risk assessment techniques. Furthermore, incorporating interactive elements and real-world scenarios can significantly improve the effectiveness of training.
Importance of Human-Centered Design in ADAS
“Human-centered design prioritizes the needs and limitations of human users when designing systems.”
Incorporating human-centered design principles into ADAS systems is essential to optimize their effectiveness and minimize potential negative impacts. This approach ensures that the technology is intuitive and complements human capabilities, rather than creating an over-reliance on systems that could lead to complacency. Designing systems that work seamlessly with the driver, providing clear and concise information, and allowing for manual overrides when needed is key.
Driver Behavior Factors and Their Relation to Accidents
Driver Behavior Factor | Relation to Accidents |
---|---|
Distraction (e.g., cell phone use) | Increased reaction time, impaired judgment, and reduced situational awareness, leading to accidents. |
Fatigue | Reduced alertness, slower reaction times, and impaired decision-making, contributing to collisions. |
Inattentiveness | Misjudged distances, incorrect lane changes, and delayed responses to hazards, increasing accident risk. |
Aggressive Driving | Imprudent lane changes, speeding, and failure to maintain safe following distances, often leading to collisions. |
Inadequate Driver Training | Lack of skills in hazard recognition, safe driving practices, and risk assessment, resulting in a higher accident rate. |
Crash Detection Algorithms and their Effectiveness
Crash detection algorithms are crucial for proactive safety systems in vehicles. These algorithms analyze sensor data to identify potential collisions, allowing for preemptive interventions like automatic braking or steering assistance. Their effectiveness is measured by accuracy, speed, and adaptability to diverse driving conditions and vehicle types.
Different Types of Crash Detection Algorithms
Various algorithms are employed for crash detection, each with its strengths and weaknesses. These range from simple threshold-based systems to more sophisticated machine learning models. Understanding the types of algorithms is essential for choosing the most suitable solution for a specific application.
- Threshold-based algorithms rely on predefined parameters to trigger an alert. For instance, exceeding a certain speed difference between vehicles or a sudden deceleration might trigger a crash warning. These algorithms are relatively simple and computationally inexpensive, making them suitable for basic crash detection systems. However, they may not be as accurate as more advanced methods, especially in complex driving scenarios.
- Rule-based algorithms employ predefined rules to evaluate sensor data. These rules might account for factors like distance, speed, and acceleration to determine if a collision is imminent. While rule-based systems can be tuned for specific driving conditions, they often require extensive rule creation and maintenance, and may not be as flexible as machine learning approaches.
- Machine learning algorithms, including neural networks and support vector machines, can learn patterns from vast datasets of sensor data. These algorithms can be trained to identify complex patterns indicative of a potential crash, leading to higher accuracy compared to other approaches. Machine learning algorithms are adaptable to various driving conditions and vehicle types, though they require substantial training data and computational resources.
Accuracy and Efficiency of Algorithms
The accuracy and efficiency of crash detection algorithms are critical factors in determining their effectiveness. Accuracy refers to the algorithm’s ability to correctly identify potential crashes, while efficiency relates to the speed and resource consumption of the detection process.
- Accuracy is often measured by precision and recall rates. A high precision rate indicates that the algorithm produces fewer false positives, while a high recall rate signifies that the algorithm correctly identifies most potential crashes. Accuracy can vary significantly depending on the algorithm type and the complexity of the driving environment.
- Efficiency is typically assessed by the algorithm’s processing time and resource utilization. Real-time crash detection requires algorithms that can analyze sensor data quickly without overwhelming the vehicle’s processing capabilities. Different algorithms have varying computational demands, impacting their suitability for embedded systems in vehicles.
Tuning Algorithms to Specific Driving Conditions
Adapting crash detection algorithms to various driving conditions is essential for robust performance. Algorithms need to be capable of adjusting their parameters based on factors like weather, traffic density, and road conditions.
- Adaptive thresholds can be implemented in threshold-based systems to adjust sensitivity based on environmental factors. For example, lower thresholds might be used in heavy rain or fog to account for reduced visibility. Similarly, more stringent thresholds might be used in high-speed scenarios.
- Machine learning models can be trained on data specific to various driving conditions. For example, training data sets could be categorized by weather, time of day, or road type to enable the model to identify patterns and make accurate predictions in different conditions.
Adapting Algorithms to Diverse Vehicle Types
Crash detection algorithms must be adaptable to accommodate the diverse range of vehicle types and sizes on the road. The sensors and data acquired will vary depending on the vehicle, and the algorithm needs to account for these differences.
- Sensor calibration is crucial to ensure consistent data across different vehicle platforms. Algorithms must be able to interpret data from different sensor types and configurations effectively.
- Model retraining might be necessary to accommodate variations in sensor data or vehicle characteristics. For example, models could be trained separately on data from different vehicle models to account for variations in sensor placement or output.
Comparison of Crash Detection Algorithms
Algorithm Type | Accuracy | Efficiency | Adaptability | Cost |
---|---|---|---|---|
Threshold-based | Moderate | High | Low | Low |
Rule-based | Moderate | Moderate | Moderate | Moderate |
Machine Learning | High | Moderate | High | High |
Safety Standards and Regulations
Safety standards play a crucial role in ensuring the effectiveness and reliability of car crash detection systems. These standards define the minimum requirements for the design, testing, and performance of advanced driver-assistance systems (ADAS), ultimately contributing to improved road safety. Regulations provide a framework for manufacturers, ensuring that ADAS systems meet established safety criteria and protect vehicle occupants and other road users.Safety standards act as a benchmark for the performance and reliability of ADAS systems.
They guarantee a minimum level of safety and performance for consumers, promoting trust and confidence in the technology. These standards are continually evolving to keep pace with advancements in technology and to address emerging safety concerns.
Role of Safety Standards in Regulating Crash Detection Systems
Safety standards define the criteria for the design, development, and testing of car crash detection systems. These criteria cover various aspects, including sensor accuracy, processing time, and the responsiveness of the system to different crash scenarios. Standards also dictate how these systems interact with other vehicle systems, ensuring a coordinated response to potentially hazardous situations. This structured approach helps maintain a high level of safety and reliability in ADAS technology.
Various Regulations and Guidelines Related to Car Crash Detection
Numerous regulations and guidelines govern the development and implementation of car crash detection systems. These include international standards, national regulations, and industry best practices. These regulations often specify the testing procedures for crash detection systems, the reporting requirements, and the procedures for compliance verification. Compliance with these regulations is crucial for manufacturers to market their products and ensures a consistent level of safety across the industry.
Importance of Testing and Certification for ADAS Systems
Thorough testing and certification are vital for ADAS systems. Rigorous testing helps identify potential weaknesses and areas for improvement in the design and functionality of these systems. Certification procedures ensure that ADAS systems meet predefined safety standards and provide a level of assurance to consumers and regulators. This process of evaluation and certification helps ensure that the system functions as intended and mitigates risks associated with its deployment.
Examples of International Standards for ADAS
Several international organizations and standards bodies contribute to the development and implementation of ADAS safety standards. Examples include ISO (International Organization for Standardization), SAE International (Society of Automotive Engineers), and various national standards bodies around the world. These standards address various aspects of ADAS systems, such as sensor accuracy, communication protocols, and system responsiveness. The consistent application of these international standards helps maintain a high level of safety across different regions and automotive markets.
Safety Standards and Their Corresponding Requirements
Safety Standard | Corresponding Requirements |
---|---|
ISO 26262 (Automotive Safety Integrity Level – ASIL) | Specifies the safety requirements for electronic/electrical systems, including crash detection systems, based on risk assessment. |
SAE J3016 | Provides guidelines for the development, testing, and evaluation of ADAS systems, focusing on functional safety. |
Euro NCAP (New Car Assessment Programme) | Evaluates vehicle safety performance, including ADAS capabilities, with specific criteria for crash avoidance. |
NHTSA (National Highway Traffic Safety Administration) | Establishes and enforces safety standards and regulations for vehicles and ADAS in the United States. |
Future Trends in Car Crash Detection and Prevention
The automotive industry is constantly evolving, driven by advancements in technology and the pressing need for enhanced safety. Future trends in crash detection and prevention are poised to dramatically improve road safety through innovative approaches and sophisticated systems. This evolution relies heavily on leveraging cutting-edge technologies, incorporating AI, and enhancing the capabilities of autonomous vehicles.The integration of advanced sensor technologies, sophisticated data processing, and intelligent algorithms is paving the way for proactive crash prevention.
Autonomous vehicles, in particular, promise a significant reduction in accidents by eliminating human error. However, the implementation of these technologies necessitates careful consideration of data privacy and security to ensure responsible deployment.
Emerging Technologies and Trends
The field is rapidly adopting new sensor technologies, such as LiDAR and radar, which provide detailed environmental data. This richer data, combined with improved processing power and sophisticated algorithms, enables more accurate and timely detection of potential hazards. Furthermore, the increased use of cameras with enhanced image processing capabilities allows for more comprehensive monitoring of the surroundings, identifying objects and pedestrians in diverse lighting conditions.
Potential of AI and Machine Learning
AI and machine learning are transforming crash detection systems. These technologies enable systems to learn from vast amounts of data, identify complex patterns, and predict potential hazards with greater accuracy than traditional methods. For instance, AI can analyze historical accident data to pinpoint common causes and develop algorithms to mitigate them. This allows for the creation of predictive models, enabling systems to anticipate potential collisions and take proactive measures.
Role of Autonomous Vehicles
Autonomous vehicles represent a significant advancement in crash prevention. Equipped with advanced sensors and sophisticated algorithms, these vehicles can detect and respond to hazards in real-time, potentially eliminating human error as a contributing factor to accidents. For example, autonomous braking systems, lane-keeping assists, and adaptive cruise control are already being integrated into vehicles, reducing the risk of collisions.
However, challenges remain in ensuring reliable and safe operation in diverse driving conditions.
Data Privacy and Security
The increasing reliance on data in crash detection systems necessitates robust measures to protect data privacy and security. Personal information collected from sensors and cameras must be handled responsibly, adhering to strict regulations and ethical guidelines. This includes implementing secure data storage, encryption protocols, and access controls. Furthermore, systems must be designed to minimize the collection of unnecessary data.
Innovative Approaches to Crash Prevention
Several innovative approaches are being explored to prevent crashes. These include the development of vehicle-to-vehicle (V2V) and vehicle-to-infrastructure (V2I) communication systems. These systems allow vehicles to share information about their location, speed, and intentions with other vehicles and infrastructure elements. This information exchange enables proactive interventions, such as coordinated braking or warning systems, to mitigate the risk of accidents.
Further innovation includes the development of advanced driver-assistance systems (ADAS) that incorporate features such as adaptive cruise control, lane-departure warnings, and automatic emergency braking, thereby enhancing the safety of drivers.
Case Studies and Real-World Applications
Real-world case studies are crucial for understanding the practical effectiveness of crash detection and prevention strategies. These examples demonstrate how technologies translate into tangible safety improvements and highlight the importance of data analysis in optimizing these systems. They also showcase the diverse applications of these strategies across various vehicle types and driving environments.
Specific Examples of Successful Crash Detection Strategies
Numerous instances demonstrate the success of proactive crash detection. For instance, advanced driver-assistance systems (ADAS) equipped with forward collision warning (FCW) and automatic emergency braking (AEB) systems have significantly reduced frontal collisions. These systems, when effectively implemented, can reduce the severity of accidents, minimize injuries, and prevent fatalities. One key example is the deployment of FCW/AEB systems in the US, which have led to a substantial decrease in certain types of crashes.
The reduction is partly due to timely alerts and automated braking responses, which prevent or mitigate collisions.
Implementation Details of Crash Prevention Technologies
The implementation of these technologies often involves several stages. Firstly, comprehensive sensor integration is crucial. Sophisticated sensors, like radar and cameras, need to be strategically placed and calibrated to ensure accurate data acquisition. Secondly, data processing and analysis pipelines need to be robust and capable of real-time processing. Accurate and rapid processing is essential for timely alerts and automated responses.
Finally, the system’s performance needs to be regularly monitored and adjusted to optimize its effectiveness in different driving conditions.
Advanced car crash detection and prevention systems are crucial, especially as the electric truck market expands. Innovations like improved sensor technology and AI-powered analysis will likely play a significant role in these systems. The integration of these technologies with the Future of electric trucks necessitates robust safety features. This focus on proactive safety measures will continue to be a key area of development for the future of vehicles.
Data Analysis for Improved Safety
Data analysis plays a critical role in optimizing crash detection and prevention systems. Statistical analysis of crash data helps identify common accident patterns and contributing factors. For example, analyzing data from vehicle sensors and driver behavior can reveal patterns that lead to collisions. This information is used to improve the algorithms and refine the response of ADAS systems, such as adjusting braking parameters or enhancing the detection of hazardous situations.
Table Summarizing Case Studies and Key Findings
Case Study | Technology Used | Key Findings | Implementation Details |
---|---|---|---|
Tesla Autopilot (FCW/AEB) | Radar, camera, and machine learning algorithms | Significant reduction in certain types of frontal collisions. | Integration of sensors into the vehicle architecture, and training of algorithms on massive datasets. |
BMW’s City Collision Mitigation System | Radar, camera, and braking system integration | Reduced rear-end collisions in low-speed scenarios. | Sophisticated algorithm integration and real-time data processing. |
Volvo’s City Safety system | Camera-based detection and braking system | Demonstrated effectiveness in preventing or mitigating low-speed collisions. | Advanced camera technology and a responsive braking system. |
Final Conclusion
In conclusion, car crash detection and prevention is a multifaceted field constantly evolving. Integrating sensor technologies, data analysis, advanced driver-assistance systems, and intelligent infrastructure will play a crucial role in making roads safer for everyone. Future research and development should focus on improving the accuracy and efficiency of these systems, as well as addressing ethical and societal implications of autonomous driving.
Answers to Common Questions
What are some common causes of rear-end collisions?
Distracted driving, following too closely, and sudden braking by the vehicle in front are common causes of rear-end collisions.
How accurate are current crash detection systems?
Accuracy varies depending on the specific system and driving conditions. Recent advancements in sensor technology and data processing have led to significant improvements in accuracy, but challenges remain in complex or unpredictable environments.
What is the role of human factors in car crashes?
Driver error, such as inattention, fatigue, or impaired judgment, is a significant contributing factor to many car crashes. Driver training and education programs play a vital role in reducing these errors.
What are the ethical considerations of autonomous vehicles in crash prevention?
Autonomous vehicles raise ethical dilemmas concerning who is responsible in case of an accident. Defining the rules of engagement in such scenarios is a critical aspect of future development and legislation.