Future of AI in business is rapidly reshaping industries, from optimizing supply chains to personalizing customer experiences. AI’s impact is already being felt across various sectors, and this transformation promises to be even more profound in the years to come. Companies are integrating AI into their operations, finding new ways to innovate, and addressing the ethical considerations that accompany this powerful technology.
This exploration delves into the multifaceted nature of AI’s role in the modern business landscape, considering its influence on existing models, workforce dynamics, and the crucial need for responsible implementation. We’ll analyze the potential benefits and challenges, from enhanced customer experiences to navigating the evolving regulatory landscape.
Impact on Existing Business Models
Artificial intelligence (AI) is rapidly transforming industries, from retail and finance to manufacturing and healthcare. This transformative power is impacting existing business models in profound ways, driving both disruption and innovation. Companies are not only adapting to AI’s influence but also actively incorporating it to enhance their operations and gain a competitive edge.The integration of AI is no longer a futuristic concept; it’s a present-day reality shaping the landscape of numerous industries.
This necessitates a shift in perspective for businesses, demanding a proactive approach to harness the potential of AI and navigate the changing market dynamics.
AI’s Transformation Across Sectors
AI is impacting various sectors through automation, data analysis, and personalized experiences. For instance, in retail, AI-powered chatbots provide 24/7 customer support, while recommendation engines personalize shopping experiences. Manufacturing is leveraging AI for predictive maintenance, optimizing production lines, and enhancing quality control. In healthcare, AI is aiding in diagnostics, drug discovery, and personalized treatment plans.
Disruption and Creation of New Business Models
AI’s potential to disrupt traditional business models is significant. Automation can lead to reduced labor costs and increased efficiency, but it also necessitates workforce reskilling and adaptation. AI-driven personalization allows businesses to tailor products and services to individual customer needs, fostering stronger customer relationships. This shift necessitates a reassessment of existing strategies and the development of innovative models.
Examples of AI Integration Success
Numerous companies have successfully integrated AI into their operations, achieving tangible results. Companies like Netflix leverage AI to recommend movies and shows, boosting user engagement and retention. Amazon utilizes AI for inventory management, supply chain optimization, and personalized recommendations, significantly improving operational efficiency. Companies like Tesla incorporate AI into their self-driving cars, pushing the boundaries of automotive technology.
Comparison of Pre-AI and Post-AI Business Models, Future of AI in business
Feature | Pre-AI Business Model | Post-AI Business Model |
---|---|---|
Customer Interaction | Limited personalized interactions, primarily through traditional channels. | Highly personalized interactions through AI-powered chatbots, virtual assistants, and customized recommendations. |
Operational Efficiency | Manual processes, potentially prone to errors and inefficiencies. | Automated processes, optimized through data analysis and predictive modeling, reducing errors and increasing efficiency. |
Decision Making | Reliance on human intuition and historical data. | Data-driven decision making leveraging AI algorithms and real-time insights. |
Product Development | Traditional product development cycles, potentially slower and less responsive to market demands. | Agile product development cycles, driven by AI-powered insights and iterative feedback loops. |
Competitive Advantage | Based on traditional strengths like brand recognition and established networks. | Based on data-driven insights, AI-powered tools, and adaptable strategies to respond to dynamic market conditions. |
AI-Driven Innovation and Development
AI is rapidly reshaping the landscape of business innovation, offering unprecedented opportunities for accelerating product development and identifying emerging market trends. This transformative technology empowers businesses to optimize processes, unlock hidden insights, and drive innovation at an unprecedented pace. The potential for AI to augment human creativity and problem-solving is significant, fostering a new era of intelligent solutions.AI’s ability to analyze vast datasets and identify patterns allows companies to gain deeper insights into customer needs and preferences.
These insights can then be used to develop innovative products and services that meet those needs more effectively. Furthermore, AI’s capacity for automation can significantly reduce development time and costs, allowing companies to bring new products to market faster.
AI’s Role in Fostering Innovation
AI is not merely an automation tool; it acts as a catalyst for innovation by enabling businesses to explore and develop entirely new ideas. By analyzing vast amounts of data, AI can identify emerging trends and unmet needs, paving the way for the creation of novel products and services. This ability to anticipate future demands is crucial in today’s dynamic market.
For example, AI can predict consumer preferences based on historical data and social media trends, enabling businesses to develop products that resonate with their target audience.
Accelerating Product Development
AI can streamline the product development lifecycle, significantly reducing time to market and improving efficiency. By automating tasks like testing, prototyping, and design, AI allows engineers and designers to focus on more creative and strategic aspects of the process. This increased efficiency can lead to faster iterations and a more agile development approach. For instance, AI-powered design tools can generate numerous design options in a fraction of the time it would take a human designer.
Identifying New Market Opportunities
AI excels at identifying previously unnoticed market opportunities. By analyzing data from diverse sources, including social media, market research reports, and customer feedback, AI can identify emerging trends, unmet needs, and potential niche markets. This allows businesses to adapt to changing consumer preferences and capitalize on emerging opportunities before competitors. For example, an AI system analyzing sales data and customer reviews might identify a need for a more eco-friendly version of a product, opening a new market segment.
Integrating AI into the Product Development Lifecycle
A strategic approach to integrating AI into the product development lifecycle is crucial for success. This involves identifying specific areas where AI can provide the most value, selecting appropriate AI tools and technologies, and ensuring seamless integration with existing systems. The following steps Artikel a structured approach:
- Data Collection and Preparation: Gather and prepare relevant data from various sources. This data should be comprehensive and representative of the target market.
- AI Model Selection and Training: Choose the most suitable AI model based on the specific needs of the product and train it on the collected data. This process often requires iterative refinement and adjustments.
- Integration into Existing Tools: Integrate the AI model into existing software tools and workflows. This step ensures seamless data flow and efficient use of AI insights.
- Continuous Monitoring and Improvement: Regularly monitor the performance of the AI model and make necessary adjustments to ensure accuracy and relevance. Feedback loops and iterative improvements are crucial.
The Future of the Workforce and Skills: Future Of AI In Business
The integration of AI into business processes is poised to reshape the job market, demanding a significant shift in workforce skills and competencies. This transformation will necessitate proactive reskilling and upskilling initiatives to ensure employees remain relevant and competitive in the evolving landscape. Adapting to this change is crucial for both individuals and organizations to thrive in the AI-driven future.AI’s impact on employment isn’t simply about job displacement, but rather a fundamental reshaping of job roles and responsibilities.
Existing roles will be augmented by AI-powered tools, requiring workers to adapt and learn new skills to leverage these technologies effectively. Furthermore, entirely new roles will emerge, catering to the management, oversight, and maintenance of these AI systems.
Potential Impact on Employment and Job Roles
The introduction of AI-driven automation will undoubtedly affect certain job roles, potentially leading to job displacement in sectors with high automation potential. However, this is not a complete loss, but a transition to new roles. Examples include data entry, repetitive manufacturing tasks, and some customer service interactions, where AI can automate many functions. Simultaneously, new roles focused on AI development, implementation, and management will emerge.
These roles will demand specific skills and expertise in areas like data science, AI ethics, and machine learning. The evolution is a crucial element to address the changing dynamics.
Emerging Skills for the AI Age
The workforce needs to adapt to the emerging requirements of an AI-driven economy. This necessitates acquiring new skills that complement AI tools and processes. Critical thinking, problem-solving, creativity, and adaptability are becoming paramount as AI handles the routine tasks. The ability to analyze data and derive insights will be a core competence. Collaboration and communication skills will also be essential to work effectively with AI systems and in diverse teams.
Strategies for Reskilling and Upskilling
Effective reskilling and upskilling programs are critical for adapting to the changing job market. Organizations must invest in training programs that equip employees with the necessary skills for the AI-driven future. This includes offering courses in data analysis, machine learning, AI ethics, and digital literacy. Individuals also have a responsibility to proactively seek out learning opportunities and invest in their own development.
Emphasis should be placed on creating a culture of continuous learning and development within the organization.
Table of Future Skills for AI-Driven Roles
Role | Essential Skills |
---|---|
AI Trainer | Machine learning, data analysis, communication, problem-solving, and project management |
AI System Analyst | Data analysis, software development, problem-solving, critical thinking, and communication |
AI Ethics Specialist | Philosophy, ethics, data privacy, legal frameworks, and communication |
Data Scientist | Statistical modeling, data mining, machine learning, programming, and communication |
AI Project Manager | Project management, communication, problem-solving, risk assessment, and stakeholder management |
Ethical Considerations and Challenges
The integration of AI into business operations necessitates a careful consideration of its ethical implications. As AI systems become more sophisticated, the potential for unintended consequences and biases must be proactively addressed. This section explores the ethical pitfalls of AI implementation, outlining potential risks and strategies for mitigation. Companies must adopt a proactive ethical framework to ensure responsible AI development and deployment.
Potential Risks and Biases
AI algorithms, trained on vast datasets, can inadvertently perpetuate and amplify existing societal biases. This can lead to discriminatory outcomes in areas like hiring, lending, and even criminal justice. For instance, facial recognition systems may exhibit lower accuracy rates for individuals from underrepresented ethnic groups, leading to misidentification and wrongful accusations. Similarly, AI-powered loan applications might unfairly deny credit to individuals from specific demographics based on biased data patterns within the training dataset.
These biases, often rooted in historical societal inequalities, can have profound and lasting negative impacts on individuals and communities.
Mitigating Ethical Concerns
A multi-faceted approach is crucial for mitigating ethical concerns associated with AI. This includes careful data selection and preprocessing to identify and remove biased data points. Furthermore, ongoing monitoring and evaluation of AI systems are vital to detect and rectify emerging biases. Transparency in AI algorithms and decision-making processes is essential to fostering trust and accountability.
Strategies for Addressing Bias
Several strategies can help mitigate bias in AI algorithms. Robust data governance and auditing procedures are essential to identify and correct potential biases in training data. Algorithmic fairness metrics should be implemented to measure and evaluate the potential for discriminatory outcomes. Regular audits and ongoing monitoring of AI systems can help identify and address any emerging biases.
Furthermore, diverse teams involved in AI development can help ensure a broader range of perspectives are considered.
Examples of Ethical AI Implementation
Several companies have proactively addressed ethical concerns in their AI development. For example, Google has implemented bias detection tools to identify and mitigate bias in their image recognition systems. They have also developed guidelines for ethical AI development that emphasize fairness, accountability, and transparency. Another example is Microsoft, which has invested in research to address fairness and bias in AI algorithms used for various applications.
These initiatives demonstrate that ethical considerations are not just theoretical concerns but are integral to the responsible development and deployment of AI in the business world.
Data Management and Security
Robust data management is crucial for any AI-driven business. The quality, quantity, and accessibility of data directly impact the accuracy, efficiency, and effectiveness of AI models. Properly managed data allows for better insights, informed decision-making, and improved operational efficiency. Furthermore, a secure data infrastructure is paramount to maintaining trust and preventing potential harm from breaches or misuse.
Importance of Data Management in AI
Data management in AI-driven businesses extends beyond simple storage. It encompasses the entire lifecycle of data, from collection and preprocessing to analysis and disposal. This includes strategies for data validation, cleaning, transformation, and version control. Effective data management ensures the integrity and reliability of the data used to train and deploy AI models, leading to more accurate and trustworthy outcomes.
A well-structured data management system fosters transparency and allows for easier audits and compliance checks.
Methods for Ensuring Data Security and Privacy
Implementing robust security measures is essential to protect sensitive data used by AI systems. These measures encompass a range of techniques, including encryption, access controls, and regular security audits. Data encryption, for instance, renders data unreadable to unauthorized individuals. Access controls restrict data access to only authorized personnel, minimizing the risk of unauthorized access. Furthermore, regular security audits identify vulnerabilities and potential weaknesses in the data management system.
Regular updates to security protocols and software are critical in countering evolving threats.
Potential Risks of Data Breaches and Vulnerabilities
Data breaches in AI systems can have significant repercussions, ranging from financial losses to reputational damage. A breach could expose sensitive customer data, leading to legal ramifications and loss of consumer trust. Furthermore, breaches can compromise intellectual property, potentially impacting the business’s competitive advantage. AI systems often rely on complex algorithms and vast datasets, making them attractive targets for malicious actors.
The financial and reputational costs associated with a breach can be substantial. The potential risks associated with vulnerabilities in AI systems are ever-evolving, requiring constant vigilance and adaptation.
Best Practices for Data Security in an AI-Driven Environment
Implementing robust security practices is crucial to mitigate risks. A strong foundation includes establishing clear data governance policies and procedures, ensuring compliance with relevant regulations, and fostering a strong security culture. Clear policies delineate the rules for data access, use, and storage. Compliance with regulations like GDPR and CCPA is mandatory to avoid legal repercussions. Fostering a security-conscious culture among employees through training and awareness programs is crucial.
This approach emphasizes the importance of data security in everyday tasks.
- Data Encryption: Encryption transforms data into an unreadable format, preventing unauthorized access. Various encryption methods exist, including symmetric and asymmetric encryption. This secures sensitive data both in transit and at rest.
- Access Control: Implementing strict access controls restricts data access to only authorized personnel. Role-based access control (RBAC) is a common approach that limits access based on user roles and responsibilities. This prevents unauthorized individuals from accessing sensitive data.
- Regular Security Audits: Regular security audits identify vulnerabilities and potential weaknesses in the data management system. Penetration testing simulates real-world attacks to assess the system’s resilience. Proactive audits can significantly reduce the risk of breaches.
- Data Loss Prevention (DLP): DLP systems monitor and prevent sensitive data from leaving the organization’s control. These systems identify and block the unauthorized transfer of sensitive information. Implementing DLP policies minimizes the risk of data leaks.
AI-Powered Customer Experience
AI is rapidly transforming customer experiences, offering businesses unprecedented opportunities to engage with customers in more meaningful and personalized ways. This evolution is driven by the ability of AI to analyze vast amounts of data, identify patterns, and anticipate customer needs, leading to more efficient and effective interactions. By understanding customer preferences and behaviors, businesses can tailor their offerings and services to better meet those needs, fostering stronger customer relationships.The application of AI extends beyond basic customer service, impacting every facet of the customer journey, from initial discovery to post-purchase support.
This transformative power is not limited to large corporations; smaller businesses can leverage AI to enhance their interactions with customers, creating a more personalized and efficient experience.
Enhancing Customer Interactions
AI-powered chatbots and virtual assistants are increasingly common in customer service. These tools can handle routine inquiries, freeing up human agents to focus on complex issues and building relationships. They can also be programmed to understand natural language, enabling more natural and efficient communication with customers.
Personalized Recommendations
AI algorithms are adept at analyzing customer data to provide highly personalized product recommendations. This approach goes beyond basic recommendations based on past purchases, leveraging factors like browsing history, social media activity, and even customer sentiment to provide more relevant and engaging suggestions. This personalization leads to increased customer satisfaction and higher conversion rates. For example, Amazon uses AI to provide tailored product recommendations, significantly increasing sales and customer engagement.
AI-Powered Customer Service Approaches
Different approaches to AI-powered customer service exist, each with its strengths and weaknesses. Rule-based systems are often used for simple queries, while machine learning models can adapt to more complex situations. Natural language processing (NLP) enables AI to understand and respond to human language, improving the conversational experience.
- Rule-Based Systems: These systems rely on predefined rules and conditions to answer customer queries. They are efficient for handling common questions but lack the adaptability of machine learning systems.
- Machine Learning Systems: These systems learn from data to improve their performance over time, allowing them to handle more complex and nuanced customer interactions. Their adaptability is a significant advantage, but they require a substantial amount of data for training.
- Natural Language Processing (NLP): NLP enables AI to understand and respond to human language in a more natural and conversational way. This technology enhances the overall customer experience by facilitating more efficient and intuitive interactions.
Improving Customer Retention and Loyalty
AI can analyze customer behavior to identify at-risk customers and proactively intervene with targeted offers or personalized support. This proactive approach to customer retention can significantly improve customer loyalty and lifetime value. Companies like Netflix use AI to identify customers who might cancel their subscriptions and offer incentives to retain them. This strategy has proven highly effective in maintaining a loyal customer base.
AI in Supply Chain Management
Artificial intelligence (AI) is rapidly transforming supply chain management, promising significant improvements in efficiency, cost reduction, and responsiveness to market fluctuations. AI’s ability to analyze vast amounts of data and learn complex patterns provides a powerful tool to optimize every stage of the supply chain, from procurement to delivery. This enhanced visibility and predictive capability allows companies to make data-driven decisions, ultimately leading to a more agile and resilient supply chain.AI’s multifaceted application in supply chain management is not limited to automation; it also encompasses advanced analytics, predictive modeling, and proactive risk mitigation.
The future of AI in business is looking bright, with significant potential for streamlining operations. This potential is directly tied to the advancements in integrated digital technologies, like those highlighted in the Integrated Digital Technologies Glendale CA A Modern Overview. Ultimately, the integration of these technologies will be crucial for companies to effectively leverage AI in the coming years.
This integration results in a more streamlined and optimized workflow, leading to better customer satisfaction and enhanced profitability.
Optimizing Supply Chain Processes
AI can significantly streamline supply chain processes by automating repetitive tasks, improving forecasting accuracy, and enhancing overall visibility. This automation reduces human error, minimizes delays, and allows companies to allocate resources more effectively. The integration of AI in various stages of the supply chain results in a more robust and adaptable system.
Predictive Analytics and Inventory Management
AI-powered predictive analytics allows companies to anticipate demand fluctuations, optimize inventory levels, and minimize stockouts or overstocking. By analyzing historical sales data, market trends, and external factors, AI algorithms can forecast future demand with greater accuracy than traditional methods. This enables companies to maintain optimal inventory levels, reducing storage costs and minimizing the risk of lost sales due to stockouts.
Real-world examples include companies like Walmart using AI to predict demand for specific products in different regions, enabling them to adjust their inventory levels accordingly and avoid costly overstocking or stockouts.
Improving Supply Chain Efficiency Using AI
AI-driven solutions can improve supply chain efficiency by enhancing visibility, optimizing transportation routes, and automating order fulfillment. AI algorithms can analyze real-time data from various sources, including logistics providers, weather forecasts, and traffic conditions, to optimize delivery routes and minimize transit times. This results in reduced transportation costs, faster delivery times, and enhanced customer satisfaction. Furthermore, AI-powered systems can automate order processing, reducing errors and improving order fulfillment speed.
AI-Driven Logistics Solutions
AI-driven logistics solutions encompass a wide range of applications, from route optimization to autonomous vehicles. AI algorithms can analyze real-time data to determine the most efficient routes for delivery trucks, considering factors such as traffic conditions, weather patterns, and delivery deadlines. This optimization can lead to significant reductions in transportation costs and delivery times. Furthermore, the emergence of autonomous vehicles, guided by AI, is poised to revolutionize the logistics industry, promising increased efficiency and safety.
Companies like Tesla and Waymo are actively developing and deploying autonomous trucking and delivery systems, which are expected to streamline logistics processes in the coming years. These systems are designed to operate 24/7, minimizing delays and improving efficiency.
Regulatory Landscape and Compliance
The rapid advancement of AI in business necessitates a robust regulatory framework to ensure responsible development and deployment. This framework must balance innovation with safeguarding consumer rights and societal well-being. The current landscape is characterized by evolving regulations and a need for clear guidelines to address the unique challenges posed by AI.The absence of a universally accepted global framework presents complexities for businesses operating internationally.
Varying legal interpretations and enforcement mechanisms across jurisdictions create a fragmented and potentially confusing regulatory environment. This dynamic situation requires companies to adapt and proactively engage with the evolving regulatory landscape.
Current Regulatory Landscape
The current regulatory landscape surrounding AI is largely composed of existing laws and regulations, not specifically designed for AI. This means that many aspects of AI development and deployment are governed by broader legal frameworks related to data privacy, consumer protection, and antitrust. Examples include GDPR in Europe, CCPA in California, and various data protection laws worldwide.
Data Privacy Compliance
Data privacy regulations, such as GDPR and CCPA, play a critical role in governing the use of AI in business. These regulations mandate that businesses handle personal data responsibly and transparently, ensuring appropriate safeguards are in place. Non-compliance can lead to significant penalties and reputational damage.
Challenges in Navigating the Legal Framework
Businesses face several challenges in navigating the legal framework surrounding AI. These include the complexity of existing regulations, the lack of specific AI-related legislation, the evolving nature of AI technology itself, and the potential for unintended consequences. Moreover, the dynamic nature of AI necessitates continuous monitoring and adaptation to ensure ongoing compliance.
AI Compliance Framework for Different Industries
Developing a comprehensive AI compliance framework requires careful consideration of the specific needs and risks of different industries. For example, the healthcare industry, with its stringent data protection requirements, must adopt a framework that aligns with HIPAA regulations, ensuring patient confidentiality and data security. The financial sector, facing the challenge of fraud detection and algorithmic bias, requires a framework encompassing regulations related to financial instruments and customer data.
Industry | Key Considerations |
---|---|
Healthcare | HIPAA compliance, patient confidentiality, data security |
Finance | Financial regulations, fraud detection, algorithmic bias, customer data protection |
Retail | Consumer data privacy, algorithmic pricing, targeted advertising |
Manufacturing | Supply chain transparency, data security, automation safety |
Examples of Potential Challenges and Solutions
A retail company using AI for targeted advertising might face challenges complying with data privacy regulations if it doesn’t have clear consent mechanisms for collecting and using customer data. Implementing a robust consent management system, transparent data usage policies, and educating customers about how their data is used can mitigate these challenges. Similarly, an autonomous vehicle company must ensure compliance with traffic regulations and safety standards while addressing the potential for liability in accidents.
The Role of Human Oversight and Collaboration
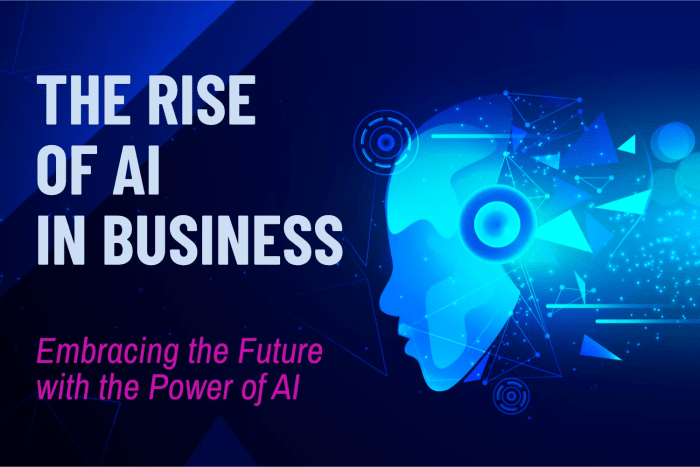
AI’s transformative potential in business hinges not on its independent operation, but on its effective integration with human expertise. Human oversight is crucial for ensuring ethical deployment, mitigating risks, and maximizing the benefits of AI systems. Collaboration between humans and AI fosters innovation and efficiency, leading to enhanced decision-making and improved outcomes.The inherent complexity of business problems often necessitates human judgment and intuition, which AI currently lacks.
By combining human insight with AI’s analytical capabilities, businesses can achieve a synergistic effect, creating opportunities for unprecedented progress.
Importance of Human Oversight in AI-Driven Businesses
Human oversight is vital for ensuring responsible AI deployment. This includes setting ethical guidelines, monitoring AI systems for bias, and rectifying any errors or unintended consequences. Humans are needed to interpret the complex outputs of AI algorithms and to apply their knowledge and experience to real-world situations. Without human oversight, AI systems can perpetuate or amplify existing societal biases, potentially leading to discriminatory outcomes.
Furthermore, human oversight provides a crucial layer of accountability and ensures that AI is used in a manner that aligns with business values and societal expectations.
Collaboration between Humans and AI
A symbiotic relationship between humans and AI is essential for success. AI excels at data analysis and pattern recognition, while humans possess critical thinking, creativity, and contextual understanding. By leveraging the strengths of both, businesses can achieve superior results. AI can automate routine tasks, freeing up human employees to focus on higher-level strategic activities. This collaborative approach fosters innovation and enables more informed decision-making.
The future of AI in business is looking bright, with innovative companies like Hikvision Digital Technology Co. Hikvision Digital Technology Co A Comprehensive Overview demonstrating how AI can be integrated into various sectors. Their focus on technological advancements suggests a promising trajectory for AI’s role in streamlining operations and boosting efficiency across industries.
Augmenting Human Capabilities with AI
AI can augment human capabilities in numerous ways. For instance, AI-powered tools can provide real-time insights and predictions, enabling quicker and more effective responses to market changes. AI can also enhance data analysis, allowing for more nuanced understanding of customer behavior and market trends. By automating repetitive tasks, AI empowers human employees to concentrate on higher-value activities like strategy development and creative problem-solving.
This augmentation leads to increased productivity and efficiency.
Examples of Human-AI Partnerships in Business
Numerous successful ventures demonstrate the power of human-AI partnerships. In the financial sector, AI-powered fraud detection systems, combined with human analysts, significantly reduce fraudulent activities. Similarly, in the healthcare industry, AI algorithms can assist medical professionals in diagnosing diseases and developing personalized treatment plans. Human oversight remains critical in interpreting these AI-generated insights and ensuring accurate and ethical application.
Moreover, in the retail sector, AI-driven recommendation engines combined with human customer service representatives create a more personalized and effective shopping experience. These examples highlight the synergistic potential of combining human expertise with AI capabilities.
End of Discussion
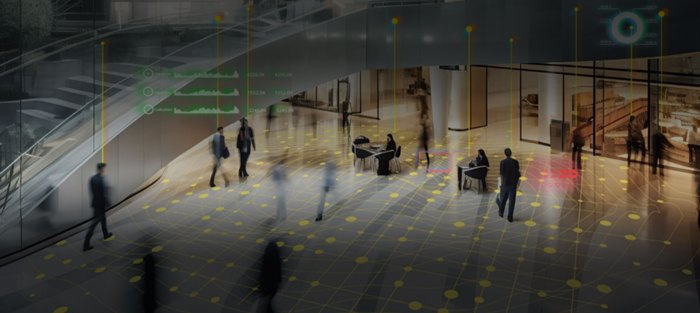
In conclusion, the future of AI in business is a dynamic and multifaceted field, demanding adaptability, ethical considerations, and strategic planning. Businesses must embrace AI’s transformative potential while carefully navigating the ethical and regulatory hurdles. The successful integration of AI hinges on a balanced approach that combines technological innovation with human oversight and a strong commitment to responsible development.
Clarifying Questions
What are some common misconceptions about AI in business?
Some believe AI will entirely replace human workers. In reality, AI augments human capabilities, creating new roles and responsibilities that demand different skillsets.
How can businesses ensure ethical AI implementation?
Businesses should prioritize fairness, transparency, and accountability in their AI systems, carefully considering potential biases and mitigating risks.
What are the key skills needed to thrive in the age of AI?
Critical thinking, problem-solving, and creativity are essential skills alongside technical expertise in AI and data analysis.
How can businesses effectively integrate AI into their existing operations?
A phased approach, starting with pilot projects and gradually expanding implementation across departments, is often the most effective strategy.