LIDAR vs. RADAR in autonomous vehicles is a crucial comparison for understanding the future of self-driving cars. Both technologies play a vital role in enabling vehicles to perceive their surroundings, but they differ significantly in their principles, capabilities, and applications. This detailed analysis explores the strengths and weaknesses of each, examining their sensing capabilities, autonomous vehicle applications, advantages and disadvantages, integration, future trends, cost considerations, environmental impact, and ethical implications.
The comparison reveals the complex trade-offs involved in choosing the right sensor technology for autonomous driving.
Introduction to LIDAR and RADAR
Autonomous vehicles rely heavily on sensors to perceive their surroundings. Two key technologies, LIDAR and RADAR, play crucial roles in enabling these vehicles to navigate safely and efficiently. Understanding their individual strengths and weaknesses is essential for evaluating their potential and limitations in autonomous driving applications.LIDAR and RADAR sensors provide different types of information about the environment. This allows autonomous vehicles to build a comprehensive understanding of their surroundings and make informed decisions.
The combination of these sensors often enhances the accuracy and reliability of the perception system, leading to safer and more capable autonomous vehicles.
LIDAR Technology
Light Detection and Ranging (LIDAR) utilizes lasers to measure the distance to objects in the environment. Pulses of laser light are emitted, and the time it takes for the reflected light to return is precisely measured. This time-of-flight measurement is then used to calculate the distance to the object. LIDAR systems can generate highly accurate 3D point clouds, providing detailed information about the shape, size, and position of objects.
RADAR Technology
Radar, or Radio Detection and Ranging, operates on a different principle. It uses radio waves to detect objects. The time delay between the transmission and reception of radio waves is measured to determine the distance to an object. RADAR systems are robust in challenging weather conditions, such as rain or fog, and can also measure the speed of objects in addition to their distance.
Comparison of Fundamental Principles
LIDAR relies on the precise measurement of light, while RADAR utilizes radio waves. LIDAR excels at creating high-resolution 3D maps of the environment, providing detailed geometric information. RADAR is more robust in adverse weather conditions and can also measure the velocity of objects, which is crucial for traffic management. The choice between LIDAR and RADAR depends on the specific requirements of the autonomous driving application.
Components of a LIDAR System
A typical LIDAR system comprises a laser emitter, a receiver, and a sophisticated data processing unit. The laser emits pulses of light, the receiver captures the reflected light, and the processing unit calculates the distance and other relevant information. Advanced LIDAR systems might also include multiple lasers, enabling simultaneous scanning from different angles.
Components of a RADAR System
A RADAR system includes a transmitter to send radio waves, a receiver to detect the reflected waves, and a signal processor to determine the distance and velocity of the target. Different RADAR systems may use different frequencies and waveforms to optimize their performance in specific environments.
Comparison Table
Feature | LIDAR | RADAR |
---|---|---|
Principle | Laser light | Radio waves |
3D Mapping | Excellent | Limited |
Weather Sensitivity | Susceptible to fog and heavy rain | Less susceptible to fog and rain |
Object Velocity Measurement | Limited | Excellent |
Cost | Generally higher | Generally lower |
Strengths | High accuracy in 3D mapping, precise object detection | Robustness in adverse weather, velocity measurement capabilities |
Weaknesses | Susceptible to environmental factors, higher cost | Limited 3D information, less precise distance measurement |
Sensing Capabilities
LIDAR and RADAR sensors are crucial for autonomous vehicles, providing vital information about the surrounding environment. These sensors enable vehicles to perceive their surroundings, identify obstacles, and make safe driving decisions. This section details the types of data collected by each sensor, their respective resolutions and accuracy, and their performance in various conditions.
LIDAR Data Collection
LIDAR (Light Detection and Ranging) sensors utilize laser pulses to measure distances to objects. The data collected consists primarily of point clouds, representing the 3D spatial coordinates of objects within the vehicle’s field of view. Each point in the cloud represents a specific location, typically with associated reflectivity data. This detailed 3D representation enables precise obstacle detection and mapping of the environment.
Furthermore, the time-of-flight technique used in LIDAR allows for accurate measurement of distances, contributing to precise object localization and understanding of the environment’s geometry.
RADAR Data Collection
RADAR (Radio Detection and Ranging) sensors operate by emitting radio waves and analyzing the reflected signals. The data gathered includes the distance, velocity, and relative position of objects. RADAR sensors provide information about the motion of objects in the environment, enabling the vehicle to predict the trajectories of moving vehicles and pedestrians. The signal strength and return time are essential for determining distance and velocity, creating an understanding of dynamic events in the surroundings.
Resolution and Accuracy
The resolution and accuracy of LIDAR and RADAR sensors significantly impact the reliability of autonomous vehicle systems. LIDAR sensors generally provide high-resolution point clouds, enabling precise representations of objects’ shapes and sizes. Accuracy depends on the specific LIDAR technology and the environmental conditions. RADAR, on the other hand, excels at detecting moving objects and determining their speed.
Accuracy is affected by factors such as signal strength and interference, influencing the reliability of the velocity and distance measurements.
Range and Detection Capabilities in Various Conditions
LIDAR sensors typically have a shorter range compared to RADAR, especially in adverse weather conditions. However, LIDAR excels in providing high-resolution 3D information, even in clear conditions. In situations with fog or rain, the reduced visibility significantly impacts the range and accuracy of LIDAR, leading to decreased detection capabilities. Conversely, RADAR is less susceptible to adverse weather, allowing for reliable detection and tracking of objects even in challenging weather conditions.
Strong sunlight can affect both LIDAR and RADAR performance.
Obstacle Detection
Both LIDAR and RADAR sensors are integral to obstacle detection systems in autonomous vehicles. LIDAR’s detailed 3D point clouds allow for precise identification of obstacles, including their shape and size. RADAR’s ability to detect moving objects and measure their speed is crucial for anticipating potential collisions. Combining the strengths of both technologies provides a comprehensive approach to obstacle detection, which improves the safety and reliability of autonomous vehicles.
Comparison Table
Feature | LIDAR | RADAR |
---|---|---|
Data Type | 3D Point Cloud (distance, reflectivity) | Distance, Velocity, Relative Position |
Resolution | High | Moderate to High (depending on the technology) |
Accuracy | High (in ideal conditions) | High (in ideal conditions) |
Range | Moderate | High |
Weather Sensitivity | Low (in clear conditions) but reduced in rain/fog | High (less affected by rain/fog) |
Obstacle Detection | Excellent for static and dynamic obstacles | Excellent for dynamic obstacles, some issues with static |
Applications in Autonomous Vehicles
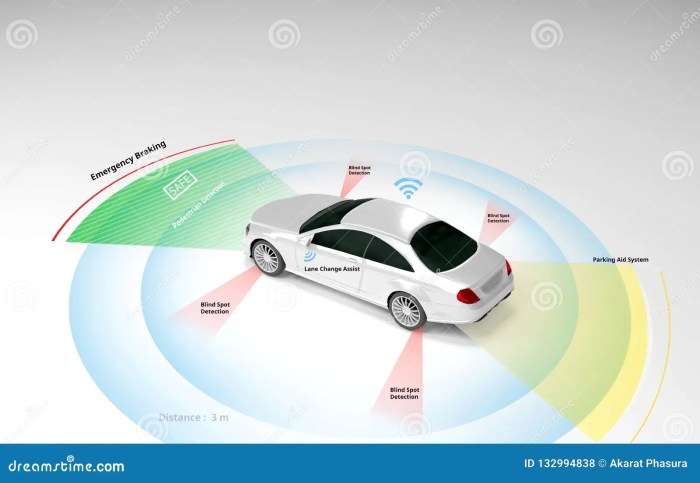
LIDAR and RADAR play crucial roles in the perception systems of autonomous vehicles, enabling them to navigate, map, and interact with their surroundings. These technologies provide complementary data, allowing for a comprehensive understanding of the environment, which is vital for safe and reliable autonomous operation. This section details how LIDAR and RADAR are employed in various autonomous vehicle functions.
LIDAR in Autonomous Vehicle Navigation
LIDAR, or Light Detection and Ranging, utilizes lasers to create high-resolution 3D maps of the environment. This technology is especially valuable for precise object detection, including their size, shape, and distance from the vehicle. By measuring the time it takes for laser pulses to reflect back, LIDAR sensors can accurately determine the distance to objects. This detailed information allows autonomous vehicles to create precise models of their surroundings, enabling safe navigation in complex environments, such as urban streets with obstacles and varied terrain.
RADAR in Autonomous Vehicle Navigation
RADAR, or Radio Detection and Ranging, relies on radio waves to detect objects. It’s particularly useful for measuring the speed and range of objects, enabling autonomous vehicles to anticipate potential collisions. While RADAR provides less detailed information about the shape and texture of objects compared to LIDAR, its ability to operate in various weather conditions makes it a crucial component of the perception system.
RADAR’s resilience to adverse weather conditions like fog and rain makes it a reliable backup to LIDAR.
LIDAR and RADAR in Mapping and Localization
LIDAR and RADAR contribute significantly to mapping and localization for self-driving cars. LIDAR’s precise 3D data creates highly detailed maps, crucial for understanding the environment and positioning the vehicle within it. RADAR, with its ability to measure speed and range, enhances localization by providing information about the relative movement of the vehicle and surrounding objects. Combining the strengths of both technologies provides a robust and accurate representation of the vehicle’s position and its surroundings.
This combined data allows for accurate mapping of dynamic environments, crucial for navigation and object recognition in diverse situations.
Integration into the Perception System
The perception system of an autonomous vehicle integrates LIDAR and RADAR data seamlessly. Data from both sensors is processed and fused to create a comprehensive understanding of the environment. Sophisticated algorithms combine the 3D point clouds from LIDAR with the speed and range data from RADAR, creating a richer and more accurate representation of the scene. This integration ensures the vehicle has a comprehensive view of its environment, allowing for reliable object detection and classification.
Role in Object Recognition and Classification
LIDAR and RADAR play distinct roles in object recognition and classification. LIDAR’s detailed 3D information is crucial for recognizing the shape and size of objects. RADAR’s speed and range data assist in classifying moving objects, such as other vehicles or pedestrians, based on their movement patterns. By combining these capabilities, the perception system can identify various objects and categorize them for safe and efficient navigation.
Summary of Applications
Autonomous Vehicle Function | LIDAR Application | RADAR Application |
---|---|---|
Navigation | Precise 3D mapping, object detection | Speed and range measurements, collision avoidance |
Mapping | High-resolution 3D maps, accurate localization | Supplementary range and speed data for dynamic environments |
Localization | Precise position determination, environmental understanding | Relative movement information, improved accuracy |
Object Recognition | Shape and size recognition, detailed object modeling | Motion analysis, moving object classification |
Perception System | Provides comprehensive 3D information | Adds crucial speed and range data, enhancing situational awareness |
Advantages and Disadvantages
LIDAR and RADAR are both crucial sensors in autonomous vehicle technology, each possessing unique strengths and weaknesses. Understanding these attributes is vital for selecting the optimal sensor suite for a specific application. Choosing the best approach often involves carefully balancing performance, cost, and environmental factors.
Advantages of LIDAR
LIDAR excels in high-precision 3D mapping and object detection. Its ability to directly measure distance, combined with its high resolution, provides unparalleled accuracy in perceiving the environment. This is particularly valuable in navigating complex, dynamic environments like urban streets.
- Precise 3D Mapping: LIDAR’s capacity for high-resolution 3D mapping allows autonomous vehicles to build detailed models of their surroundings. This detailed understanding is essential for navigation and object recognition, enabling vehicles to safely navigate intricate environments, such as dense urban areas, and identify subtle obstacles like pedestrians or cyclists.
- High Accuracy: LIDAR’s direct distance measurement leads to extremely accurate object localization and classification. This accuracy is crucial for autonomous vehicles to react promptly and precisely to changing conditions, enabling safe maneuvers in various situations. For instance, it can precisely determine the position of a parked car, vital for avoiding collisions.
- Strong Performance in Various Conditions: LIDAR, especially with advancements in technology, performs well in various weather conditions, including fog and rain, although its performance can still be affected by certain adverse conditions. This robustness is a significant advantage compared to other sensor types.
Advantages of RADAR
RADAR is a robust sensor, especially in challenging environmental conditions. Its ability to penetrate certain materials and operate in varying weather conditions provides a distinct advantage.
- Robustness in Challenging Conditions: RADAR’s ability to function in rain, fog, and other adverse weather conditions makes it a dependable sensor for autonomous vehicles operating in diverse climates. This reliability is especially important for vehicles operating in regions with unpredictable weather patterns.
- Long-Range Detection: RADAR excels at detecting objects at longer distances than LIDAR. This capability is essential for maintaining safe distances and reacting to obstacles in extended driving situations.
- Cost-Effectiveness: Compared to LIDAR, RADAR systems often have a lower cost, making them more accessible for integration into autonomous vehicle platforms.
Disadvantages of LIDAR
While LIDAR possesses significant strengths, it also faces some limitations.
- High Cost: LIDAR sensors are often more expensive than RADAR sensors. This higher cost can be a significant factor in the overall cost of autonomous vehicles.
- Limited Range: LIDAR’s range for accurate detection is generally shorter than that of RADAR, especially in challenging conditions. This can be a concern in long-distance driving or when navigating environments with sparsely distributed objects.
- Susceptibility to Interference: LIDAR can be affected by environmental factors, such as sunlight glare, which can negatively impact its accuracy and detection capabilities.
Disadvantages of RADAR
RADAR also has some limitations.
- Limited 3D Information: RADAR primarily provides 2D information, unlike LIDAR, which offers 3D data. This limitation can reduce the accuracy of object recognition and localization in certain scenarios.
- Difficulty in Identifying Subtle Features: RADAR may struggle to distinguish subtle features or textures of objects, which can be crucial for accurate object classification. This might lead to potential misclassifications in certain scenarios.
- Sensitivity to Weather: While generally robust, RADAR’s performance can be impacted by very heavy rain or extremely high humidity, although this is often less severe than the effect on LIDAR.
Trade-offs Between LIDAR and RADAR
The selection between LIDAR and RADAR depends on the specific needs of the application. A combination of both sensors often provides the most robust solution.
LIDAR and RADAR are crucial for autonomous vehicle navigation, but the future of AI in business, like in self-driving cars, hinges on these technologies’ development. Future of AI in business will likely see AI-powered systems optimizing everything from supply chains to customer service, mirroring the advancements needed for accurate LIDAR and RADAR fusion in autonomous vehicles. The ongoing debate about which technology is superior for perception remains a key area of research in this field.
- Cost vs. Performance: RADAR often presents a more cost-effective option, but its performance limitations may necessitate complementary LIDAR systems for high-accuracy applications. The choice depends on whether the budget allows for the cost of higher-performance LIDAR or whether a less expensive RADAR is sufficient.
- Environmental Conditions: RADAR’s robustness in adverse weather conditions makes it suitable for areas with high variability in weather. However, LIDAR’s superior 3D perception in clear conditions can be a necessity in certain applications.
Comparison Table
Feature | LIDAR | RADAR |
---|---|---|
3D Information | High | Low |
Range | Medium | High |
Accuracy | High | Medium |
Cost | High | Low |
Weather Robustness | Medium | High |
Integration and Data Fusion: LIDAR Vs. RADAR In Autonomous Vehicles
Integrating LIDAR and RADAR data is crucial for enhancing the perception capabilities of autonomous vehicles. Combining these complementary sensor modalities allows for a more comprehensive understanding of the surrounding environment, leading to more robust and reliable decision-making. This fusion process, while presenting challenges, is vital for achieving safe and efficient autonomous operation.
Data Fusion Process Overview
The process of integrating LIDAR and RADAR data involves combining the individual sensor readings to create a more complete and accurate representation of the environment. This entails transforming the data into a common coordinate system, aligning the measurements, and then combining the information. Crucially, the fusion algorithm needs to address potential inconsistencies or discrepancies in the data. Sophisticated algorithms are designed to reconcile these differences, thereby providing a more holistic perception of the environment.
Methods for Handling Data Discrepancies, LIDAR vs. RADAR in autonomous vehicles
LIDAR and RADAR sensors often provide different perspectives and levels of detail. For instance, LIDAR excels at precise 3D object modeling, while RADAR is superior at detecting objects at longer ranges and through weather conditions. Addressing these discrepancies requires sophisticated algorithms. One approach is to prioritize sensor data based on reliability metrics, assigning higher weight to data deemed more accurate.
Another strategy is to use a probabilistic approach, where the likelihood of a particular object or feature being present is calculated based on the readings from both sensors. Techniques like Kalman filtering are frequently used to smooth and interpolate data, mitigating inconsistencies and improving overall accuracy.
Data Fusion Techniques
Various data fusion techniques are employed in autonomous vehicles. These techniques range from simple data averaging to more sophisticated algorithms.
- Data Averaging: This is a basic method where the readings from both LIDAR and RADAR are averaged to generate a combined measurement. While simple, it can be insufficient for complex scenarios where the sensors have significant differences in their readings. This method can work well for basic tasks but isn’t suitable for complex situations.
- Bayesian Filtering: This technique uses probabilistic models to estimate the likelihood of different interpretations of the environment. It updates these estimates as new data arrives from either sensor. Bayesian filtering is computationally intensive but is often used for scenarios with high uncertainty.
- Kalman Filtering: This method is widely used to estimate the state of a dynamic system. It combines measurements from LIDAR and RADAR to improve the accuracy and reliability of the estimated state. Kalman filtering excels in scenarios involving moving objects and changing conditions.
- Neural Networks: Advanced neural network architectures can learn complex relationships between LIDAR and RADAR data. These methods can be very effective in identifying patterns and making predictions in complex environments. Their high performance comes with a trade-off of high computational demands.
Illustrative Example of Data Fusion
Imagine an autonomous vehicle encountering a pedestrian crossing the road. LIDAR provides a detailed 3D model of the pedestrian, while RADAR detects their presence at a longer range, perhaps even before the pedestrian enters the vehicle’s field of view. By fusing the data, the vehicle can accurately estimate the pedestrian’s trajectory, speed, and intentions, leading to a more informed and appropriate response.
Table of Data Fusion Techniques
Technique | Description | Advantages | Disadvantages |
---|---|---|---|
Data Averaging | Simple average of LIDAR and RADAR measurements. | Easy to implement. | Ineffective for scenarios with significant differences between sensors. |
Bayesian Filtering | Probabilistic approach to data fusion. | Handles uncertainty effectively. | Computationally intensive. |
Kalman Filtering | Estimates the state of a dynamic system. | Effective for tracking moving objects. | Requires accurate model of the system. |
Neural Networks | Learns complex relationships between sensor data. | High accuracy in complex scenarios. | Requires large datasets for training. |
Future Trends
The autonomous vehicle landscape is constantly evolving, driven by advancements in sensor technology. LIDAR and RADAR are crucial components, and their future development promises to significantly enhance the capabilities and safety of self-driving cars. These advancements will be pivotal in creating more robust, reliable, and safer autonomous vehicle systems.Future iterations of these technologies will be critical for achieving widespread adoption of autonomous vehicles, paving the way for a more efficient and potentially safer transportation future.
Potential Advancements in LIDAR Technology
LIDAR technology is poised for significant advancements, particularly in terms of range, resolution, and cost-effectiveness. Miniaturization of components and improved manufacturing processes are anticipated, leading to more compact and affordable LIDAR systems. Further development in solid-state LIDAR is expected to reduce power consumption and increase reliability. The integration of AI algorithms with LIDAR data will be crucial in extracting more meaningful information from the sensor data.
This could include advanced object classification, improved 3D modeling, and enhanced environmental understanding, ultimately contributing to a more sophisticated and adaptable perception system.
Potential Advancements in RADAR Technology
RADAR technology is also expected to see significant improvements in the coming years. One key area is the development of higher-frequency RADAR sensors, enabling improved resolution and differentiation between objects. This will allow for more accurate and precise object detection, even in challenging conditions. Another focus is on developing RADAR systems capable of operating in a wider range of environmental conditions, including adverse weather and complex urban environments.
This involves enhancing the robustness and reliability of RADAR systems in challenging conditions. Researchers are also investigating the use of multi-frequency RADAR, enabling better object characterization and classification.
Development of More Sophisticated and Efficient Sensors
Future autonomous vehicles will likely incorporate more sophisticated and efficient sensors beyond LIDAR and RADAR. Researchers are exploring the use of hyperspectral imaging and thermal imaging to supplement existing sensor data. Hyperspectral imaging, capable of capturing the spectral signature of objects, can improve object identification and classification. Thermal imaging can be helpful in detecting heat signatures, enabling the detection of objects in low-light conditions and aiding in distinguishing between objects with similar shapes.
This combined approach will significantly enhance the overall perception and understanding of the environment by the autonomous vehicle.
Possibilities of Hybrid Systems Combining LIDAR and RADAR
The combination of LIDAR and RADAR technologies offers a compelling approach to enhance autonomous vehicle perception. Hybrid systems, leveraging the strengths of both technologies, can create a more comprehensive understanding of the environment. LIDAR excels in high-resolution 3D mapping and object detection in clear weather, while RADAR offers penetration capabilities in challenging weather conditions. The fusion of this data allows for a more reliable and robust perception system, crucial for safety and reliability in diverse environments.
An example of a hybrid system would be one that uses LIDAR to create a detailed map of the environment during clear conditions and uses RADAR to supplement this map during adverse weather, enabling more comprehensive environmental awareness.
Examples of Future Advancements in LIDAR and RADAR Improving Autonomous Vehicle Safety
Advancements in LIDAR and RADAR will significantly improve autonomous vehicle safety. For instance, enhanced object detection and classification capabilities will allow autonomous vehicles to better distinguish between pedestrians, cyclists, and other vehicles, leading to improved reaction times and reduced accident risks. The ability of RADAR to operate effectively in challenging weather conditions, such as heavy rain or fog, will enable autonomous vehicles to safely navigate in these conditions, reducing the risk of accidents.
Furthermore, more precise localization and mapping capabilities, enabled by advancements in both technologies, will contribute to more reliable and safer navigation systems.
Cost Considerations
The economic viability of implementing LIDAR and RADAR systems in autonomous vehicles is a critical factor influencing their widespread adoption. Understanding the cost structures and associated factors is essential for evaluating the long-term feasibility of these technologies. Comparing the costs and potential return on investment is vital for developers and manufacturers.The cost of implementing LIDAR and RADAR systems varies significantly, impacting the overall price of autonomous vehicles.
Factors like sensor technology, processing power, and integration complexity play a substantial role in determining the final cost. The choice between LIDAR and RADAR, or a combination of both, heavily depends on the desired balance between cost and performance.
Cost Comparison of LIDAR and RADAR Systems
LIDAR sensors, while often providing higher accuracy and detailed 3D information, generally command a higher price tag compared to RADAR. This difference stems from the more complex and specialized components within LIDAR systems. Furthermore, the higher computational demands of processing LIDAR data can also contribute to increased costs. Conversely, RADAR systems, typically employing more mature technology, are often less expensive to manufacture and maintain.
However, RADAR’s range and precision capabilities are often less impressive than those of LIDAR.
Factors Influencing Sensor Costs
Several factors significantly influence the cost of LIDAR and RADAR sensors. Sensor resolution, range, and accuracy directly impact production costs, as higher performance specifications usually require more sophisticated components. The manufacturing process, including material costs and assembly complexity, also plays a role. Furthermore, economies of scale can substantially influence pricing; as production volumes increase, the unit cost of components tends to decrease.
The sophistication of the accompanying electronics, such as signal processing units, is also a crucial factor.
Impact of Cost on Adoption Rate
High initial costs often serve as a significant barrier to the adoption of LIDAR and RADAR systems in autonomous vehicles. This factor, coupled with the need for substantial infrastructure upgrades, often delays widespread adoption. However, as the technology matures and manufacturing processes improve, costs are anticipated to decrease, potentially leading to a more rapid uptake of these technologies.
The decreasing costs are a key driving force for greater adoption, particularly for consumer-level autonomous vehicles.
Cost Implications of Data Fusion
Data fusion, the process of combining information from multiple sensors like LIDAR and RADAR, is crucial for achieving robust and reliable autonomous vehicle perception. However, data fusion adds to the complexity and computational load of the system, potentially leading to increased costs. Advanced algorithms and sophisticated processing units are necessary for effectively merging the data from different sensors.
This increased complexity translates into higher development costs, but can also lead to enhanced safety and performance, potentially justifying the investment.
Cost-Benefit Analysis of LIDAR and RADAR Systems
A thorough cost-benefit analysis for LIDAR and RADAR systems in autonomous vehicles requires a comprehensive assessment of potential savings and risks. Factors such as enhanced safety, improved performance, reduced operational costs, and potential market advantages should be considered alongside the initial investment and maintenance expenses. The benefits of improved safety and reduced accident rates could significantly outweigh the costs, particularly in the long run.
Environmental Impact
Autonomous vehicles, promising a future of efficient and potentially safer transportation, also present a range of environmental considerations. The manufacturing processes, energy consumption, and overall lifecycle of both LIDAR and RADAR systems have implications for resource use and waste generation. A thorough understanding of these impacts is crucial for evaluating the long-term sustainability of these technologies.
Manufacturing Impacts of LIDAR Sensors
The production of LIDAR sensors involves the use of various materials, including lasers, detectors, and optical components. Manufacturing these components requires significant energy and raw materials, potentially contributing to resource depletion and pollution. For instance, the production of gallium arsenide used in some laser diodes is an energy-intensive process, impacting energy consumption and potentially contributing to environmental issues like greenhouse gas emissions.
Additionally, the disposal of manufacturing waste and the potential for hazardous materials used in the manufacturing process need careful consideration.
Choosing between LIDAR and RADAR for autonomous vehicles is a bit like deciding between Agile and Waterfall development methodologies. Just as Agile emphasizes flexibility and iterative improvements, LIDAR often offers a more dynamic and adaptable approach to perception. This is particularly useful when dealing with complex and unpredictable environments. Conversely, RADAR, like Waterfall, provides a more structured and predictable solution, but may lack the agility to quickly adapt to evolving conditions.
Ultimately, the optimal choice depends heavily on the specific needs and constraints of the autonomous vehicle application. Agile vs. Waterfall demonstrates this trade-off clearly in software development, highlighting how both methodologies can be valuable, depending on the context. This parallels the situation in autonomous vehicles, where the best sensor technology depends on the application.
Manufacturing Impacts of RADAR Sensors
Similar to LIDAR, the production of RADAR sensors necessitates energy and raw materials. Key components like the transceiver modules, antennas, and microcontrollers all have associated environmental impacts during manufacturing. The specific materials used and their manufacturing processes will vary depending on the type of RADAR sensor, influencing the environmental footprint. For example, the extraction and processing of certain metals used in the components might contribute to land degradation and pollution.
Energy Consumption of LIDAR and RADAR Systems
The operational energy consumption of LIDAR and RADAR systems is a critical aspect of their environmental impact. LIDAR systems, relying on lasers, typically consume more energy than RADAR systems. This energy consumption, often drawn from the electrical grid, contributes to greenhouse gas emissions depending on the source of electricity. The power requirements of the systems can also vary depending on the complexity of the sensor and the surrounding environment.
Sustainability Concerns
Several sustainability concerns are associated with both LIDAR and RADAR technologies. The finite nature of certain raw materials used in their manufacture raises concerns about resource depletion. The energy consumption during operation, particularly for high-resolution LIDAR systems, could increase the carbon footprint of autonomous vehicles. Furthermore, the lifecycle assessment of these systems, encompassing manufacturing, operation, and eventual disposal, needs to be thoroughly examined to identify potential environmental hotspots and implement sustainable practices.
Lifecycle Assessment of LIDAR and RADAR Systems
A lifecycle assessment (LCA) of LIDAR and RADAR systems examines the environmental impacts throughout their entire life cycle. This includes material sourcing, manufacturing processes, transportation, use, and eventual disposal. LCA studies for these technologies are still ongoing, but they can help identify the environmental hotspots in the production and use of these technologies. By understanding the different stages of the lifecycle, potential environmental impacts can be minimized through design improvements and material choices.
For instance, choosing materials with lower embodied energy or implementing energy-efficient manufacturing processes can reduce the overall environmental footprint.
Ethical Considerations
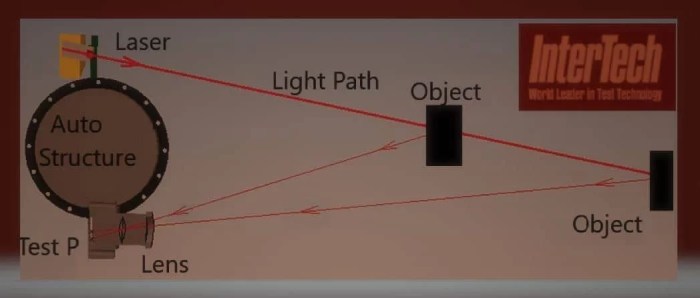
Autonomous vehicles, relying heavily on LIDAR and RADAR, present a unique set of ethical dilemmas. These systems, while promising advancements in safety and efficiency, introduce complexities regarding decision-making in unavoidable accident scenarios. The potential for bias in data collection and security vulnerabilities also warrant careful consideration. Understanding these ethical implications is crucial for responsible development and deployment of these technologies.
Potential Biases in Data Collection
LIDAR and RADAR sensors, while providing valuable data, can reflect inherent biases present in the data sets used for training algorithms. For example, if the majority of training data comes from one geographic region or demographic, the system might perform poorly in other environments or for different user groups. This can lead to discrepancies in performance and potentially discriminatory outcomes, such as varying levels of safety or responsiveness to different types of drivers or pedestrians.
Security Concerns
Autonomous vehicles are susceptible to hacking and manipulation, potentially jeopardizing the safety of passengers and others on the road. Vulnerabilities in the software and hardware systems used in LIDAR and RADAR could be exploited to cause unexpected or dangerous behavior. Furthermore, the data collected by these sensors, including detailed maps of surroundings and driver behavior, could be vulnerable to theft or misuse, raising significant privacy concerns.
Ensuring robust security protocols and data encryption is essential for maintaining the integrity and safety of these systems.
Societal Impact
The widespread adoption of autonomous vehicles could have a profound societal impact. Job displacement in transportation-related industries is a concern, requiring careful consideration and proactive strategies for workforce adaptation. The potential for increased accessibility for individuals with disabilities, however, is a significant advantage. These vehicles also pose questions about liability in accident scenarios, requiring clear legal frameworks to address these issues.
Examples of Ethical Autonomous Vehicles
Companies are exploring innovative approaches to mitigate these concerns. Developing robust algorithms that account for diverse environments and scenarios is crucial. Furthermore, integrating ethical frameworks into the development process to address decision-making in complex situations, like unavoidable accidents, is important. Open-source platforms that facilitate the sharing of data and algorithms could help in achieving better performance and mitigate bias in the long run.
Last Word
In conclusion, both LIDAR and RADAR offer unique advantages for autonomous vehicles, with LIDAR excelling in high-resolution object detection and RADAR demonstrating robustness in challenging weather conditions. The optimal solution often lies in integrating both technologies for a comprehensive perception system, enabling more reliable and robust decision-making. Future developments promise even more sophisticated and efficient sensors, paving the way for safer and more reliable autonomous vehicles.
However, factors like cost, environmental impact, and ethical considerations must be carefully evaluated to ensure responsible development.
FAQ Resource
What are the key differences in the data collected by LIDAR and RADAR sensors?
LIDAR provides precise 3D point cloud data, crucial for detailed object shape and distance information. RADAR, on the other hand, excels at detecting movement and range, offering robust performance in challenging weather conditions, though it typically provides less detailed shape information.
How does the cost of LIDAR compare to RADAR?
LIDAR sensors generally have a higher initial cost compared to RADAR, though advancements are constantly reducing the gap. The long-term cost implications, including maintenance and potential for data fusion, should also be considered.
What are the environmental impacts of these technologies?
Both LIDAR and RADAR systems have environmental implications related to manufacturing, energy consumption, and potential waste disposal. The environmental impact of each technology needs further investigation, considering the entire lifecycle of the sensor.
What are some of the potential ethical considerations in using LIDAR and RADAR?
Ethical considerations include potential biases in the data collected by sensors, the need for safe and responsible autonomous vehicle design, and the impact on employment in the transportation industry. These issues must be addressed as autonomous vehicle technology advances.