With The role of AI in car safety at the forefront, this exploration dives deep into how artificial intelligence is transforming the automotive industry. From driver-assistance systems to fully autonomous vehicles, AI is rapidly changing how we interact with and experience the act of driving. This comprehensive look reveals the potential benefits and challenges of this technological leap.
The introduction provides a concise overview of the use of AI in automotive safety, examining the different AI algorithms employed in autonomous driving and safety features. A historical context is established, tracing the evolution of AI in the automotive industry. A comparison table illustrates the distinctions between traditional safety systems and their AI-powered counterparts.
Introduction to AI in Automotive Safety
Artificial intelligence (AI) is rapidly transforming the automotive industry, with a significant focus on enhancing vehicle safety. AI algorithms are being integrated into various systems, from autonomous driving to traditional safety features, leading to a new era of accident prevention and driver assistance. This evolution is driven by the potential of AI to analyze vast amounts of data and make real-time decisions, surpassing human capabilities in certain situations.AI’s role in automotive safety encompasses a wide range of applications, including advanced driver-assistance systems (ADAS), autonomous driving, and predictive maintenance.
These systems leverage different types of AI algorithms to achieve enhanced safety, from image recognition to machine learning for decision-making.
Types of AI Algorithms Used in Automotive Safety
AI algorithms are integral to the functionality of modern safety systems. Different algorithms are used depending on the specific task. Supervised learning, a common approach, trains algorithms on labeled data to identify patterns and make predictions. Unsupervised learning, conversely, finds hidden patterns in unlabeled data, allowing for anomaly detection and improved system performance. Reinforcement learning is crucial for autonomous vehicles, allowing the system to learn through trial and error and refine its decision-making processes.
Historical Progression of AI in Automotive
The integration of AI into automotive safety has a clear historical progression. Early systems focused on simple sensor-based functions, such as anti-lock brakes (ABS). Over time, the complexity and sophistication of these systems increased, incorporating more advanced algorithms and sensors. The advent of machine learning enabled the development of systems capable of handling complex scenarios and improving safety outcomes in various driving conditions.
Today, the development of AI is enabling more autonomous driving features and predictive maintenance capabilities.
Comparison of Traditional and AI-Powered Safety Systems
Feature | Traditional Safety Systems | AI-Powered Safety Systems | Key Differences |
---|---|---|---|
Obstacle Detection | Simple sensors (e.g., radar, cameras) for detecting objects in the immediate vicinity. | Sophisticated computer vision and deep learning models for recognizing and classifying objects, including pedestrians, cyclists, and other vehicles, at a distance. AI can analyze context, such as traffic flow and road conditions, for more nuanced decisions. | AI systems provide more comprehensive and contextual awareness, allowing for anticipatory actions. |
Decision Making | Pre-programmed responses based on predefined rules and conditions. | Adaptive decision-making based on real-time data analysis and learned patterns. AI can adjust responses based on the dynamic environment. | AI allows for dynamic adaptation to changing conditions, exceeding the capabilities of pre-programmed responses. |
Predictive Maintenance | Routine checks and maintenance based on pre-determined schedules. | Predictive maintenance models using machine learning to forecast potential vehicle failures based on sensor data and historical patterns. | AI-powered systems anticipate potential issues, reducing downtime and improving overall safety. |
Adaptability | Limited adaptability to changing conditions. | High adaptability to diverse driving conditions and unexpected situations. | AI systems are more adaptable to varying conditions, allowing for a more robust safety approach. |
AI-Powered Driver-Assistance Systems
AI-powered driver-assistance systems are rapidly transforming the automotive landscape, offering enhanced safety and convenience. These systems leverage machine learning algorithms to interpret complex data from various onboard sensors, enabling proactive responses to potential hazards. From adaptive cruise control to lane-keeping assist, these technologies aim to reduce human error and enhance overall driving experience.Driver-assistance systems are increasingly sophisticated, integrating multiple functionalities to create a safer driving environment.
These systems rely on intricate sensor networks, including cameras, radar, and lidar, to perceive and react to their surroundings. The analysis and processing of this data by AI algorithms form the foundation of these systems’ capabilities.
Adaptive Cruise Control
Adaptive cruise control (ACC) automatically adjusts a vehicle’s speed to maintain a safe following distance from the vehicle ahead. This system uses radar or lidar to detect the distance to the preceding car and automatically adjusts the vehicle’s speed to maintain the set following distance. This feature is designed to mitigate the risk of rear-end collisions, particularly in heavy traffic.
Lane Departure Warning
Lane departure warning (LDW) alerts the driver if the vehicle deviates from its lane unintentionally. This system utilizes cameras to monitor the lane markings on the road and provides a visual or auditory alert to the driver if the vehicle is drifting out of its lane. This feature is designed to help prevent accidents caused by unintentional lane drifting.
Autonomous Emergency Braking
Autonomous emergency braking (AEB) is a crucial safety feature that automatically applies the brakes to prevent collisions in certain situations. The system uses radar or cameras to detect potential collisions with vehicles or pedestrians and automatically applies the brakes to mitigate or avoid the collision. AEB systems vary in their sophistication, from basic systems that react to potential collisions with other vehicles to more advanced systems that can detect pedestrians and cyclists.
Other Driver-Assistance Systems
Other advanced driver-assistance systems include blind-spot monitoring, rear cross-traffic alert, and automatic parking assist. These systems use a combination of sensors to enhance the driver’s awareness of their surroundings and help to mitigate potential hazards. Blind-spot monitoring systems use radar to detect vehicles in the driver’s blind spots, alerting the driver with visual or auditory signals. Rear cross-traffic alert systems help drivers avoid collisions with vehicles approaching from the side when backing up.
Automatic parking assist systems guide the vehicle into parking spaces, simplifying the parking process.
Limitations and Potential Risks
While these systems offer significant benefits, limitations and potential risks exist. For instance, the performance of these systems can be affected by adverse weather conditions or poor visibility. Malfunctions in the sensor systems or AI algorithms could lead to erroneous warnings or inaction. Over-reliance on these systems could diminish the driver’s alertness and proactive decision-making. A critical aspect is the need for constant maintenance and updates to ensure the optimal functioning of the systems.
Comparison of Effectiveness Across Models
The effectiveness of AI-driven driver-assistance systems varies across different car models. Factors such as the quality and resolution of sensors, the sophistication of the AI algorithms, and the integration of different systems influence the performance of these systems. Comparative studies and real-world testing data are crucial to evaluate the performance of these systems in various driving conditions.
AI plays a crucial role in enhancing car safety, especially in the context of Autonomous & Self-Driving Cars Autonomous & Self-Driving Cars. These vehicles rely heavily on AI algorithms for tasks like object detection and decision-making in complex scenarios. Ultimately, advancements in AI are vital to improving the overall safety of automobiles, regardless of their level of automation.
Table of Driver-Assistance Systems
Feature | Benefits | Potential Drawbacks | Effectiveness (General Assessment) |
---|---|---|---|
Adaptive Cruise Control | Maintains safe following distance, reduces driver fatigue, and improves fuel efficiency. | May not adapt effectively in complex traffic situations or with sudden changes in the road conditions. | Good, but depends on the specific implementation. |
Lane Departure Warning | Alerts drivers to potential lane departures, reducing the risk of accidents. | False alarms can occur, and drivers may become overly reliant on the system. | Generally effective, but requires careful calibration. |
Autonomous Emergency Braking | Potentially prevents collisions with other vehicles and pedestrians. | Performance can vary depending on the complexity of the situation, and the system’s ability to accurately interpret the environment. | Promising, but needs further refinement. |
Blind-Spot Monitoring | Increases driver awareness of vehicles in blind spots, enhancing safety. | Can sometimes trigger false alerts, potentially causing driver distraction. | Good, especially for avoiding lane-changing accidents. |
Autonomous Driving and Safety
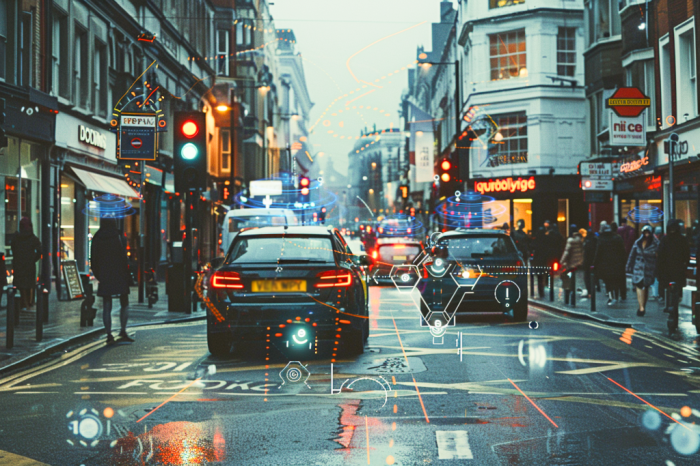
Autonomous vehicles promise a future of safer, more efficient transportation. However, achieving true safety in fully autonomous driving presents unique challenges. These systems, reliant on sophisticated AI, must navigate complex scenarios and make critical decisions in real-time, surpassing the capabilities of human drivers in some situations. The development and deployment of these technologies demand meticulous attention to safety protocols and rigorous testing.
The Role of AI in Autonomous Decision-Making
AI plays a crucial role in enabling autonomous vehicles to perceive their surroundings and make decisions. Sensor fusion, combining data from various sources like cameras, radar, and lidar, allows the vehicle to create a comprehensive understanding of the environment. Sophisticated algorithms then analyze this data to predict the behavior of other vehicles, pedestrians, and obstacles. This data-driven analysis guides the vehicle in selecting appropriate maneuvers, such as braking, accelerating, or steering.
The system’s decision-making process must be robust and capable of adapting to diverse conditions.
Safety Challenges in Autonomous Vehicles
Autonomous vehicles face several unique safety challenges. Unforeseen or complex situations, such as unexpected pedestrian actions or malfunctioning sensors, can lead to difficult decision-making scenarios. Ensuring the reliability and robustness of the AI systems in the face of these unpredictable circumstances is paramount. Accidents caused by AI errors can have severe consequences, highlighting the critical need for rigorous testing and validation of these systems in diverse conditions.
Levels of Autonomous Driving and Safety Features
Different levels of autonomous driving capabilities exist, each with its corresponding safety features. Level 0 vehicles require human intervention at all times. In contrast, Level 5 vehicles operate autonomously without any human input. The increasing autonomy levels necessitate a corresponding evolution in safety features. For instance, Level 2 vehicles often include adaptive cruise control and lane keeping assistance, whereas Level 3 vehicles may include partial driver-monitoring systems.
These features are designed to assist the driver and mitigate risks, but ultimately, the driver retains control. Higher levels of autonomy demand more sophisticated safety features to handle more complex situations.
Decision-Making Flowchart in Critical Situations
Start -->
|
V
Sensor Data Acquisition (Cameras, Lidar, Radar) -->
|
V
Data Fusion & Processing -->
|
V
Situation Assessment (Pedestrian, Vehicle, Obstacle Detection) -->
|
V
Risk Evaluation (Probability of Collision, Severity) -->
|
V
Decision Selection (Braking, Steering, Acceleration, Avoidance Maneuvers) -->
|
V
Execution of Selected Maneuver -->
|
V
Feedback & Adjustment -->
|
V
End
This flowchart Artikels the decision-making process within an autonomous vehicle during a critical situation. It emphasizes the systematic nature of the process, from initial sensor input to the final execution of the chosen maneuver.
AI for Accident Avoidance and Mitigation
AI is increasingly crucial in proactively preventing accidents in the automotive industry. By leveraging sophisticated algorithms and vast datasets, AI systems can analyze complex situations in real-time, predicting potential hazards and triggering preventative measures to mitigate risks. This proactive approach to safety represents a significant advancement in automotive technology.
Predictive Accident Prevention
AI systems can analyze a multitude of data points, including road conditions, weather patterns, traffic flow, and driver behavior, to identify potential accident precursors. Sophisticated machine learning models are trained on massive datasets of historical accident reports, allowing them to identify subtle patterns and correlations that human analysts might miss. This predictive capability allows AI to alert drivers or intervene automatically to prevent accidents.
For example, a system might anticipate a sudden braking maneuver by another vehicle and adjust the car’s speed accordingly.
AI-Driven Collision Avoidance Systems
Numerous AI-driven systems are being developed to prevent collisions. Adaptive cruise control, which automatically adjusts speed to maintain a safe following distance, is a well-established example. Advanced systems go beyond this by using cameras and sensors to detect potential obstacles and react accordingly. Autonomous emergency braking (AEB) systems, for instance, can automatically apply the brakes if a collision is imminent.
These systems are crucial for preventing accidents in various scenarios, such as sudden stops or lane changes.
Accident Reconstruction and Analysis
AI plays a valuable role in accident reconstruction and analysis. By analyzing data from various sources, including sensor data from the vehicles involved, witness statements, and traffic camera footage, AI can create a detailed account of the accident. This can be instrumental in determining the cause of the accident, which in turn aids in preventing similar accidents in the future.
For example, AI can identify common patterns in accidents occurring at specific intersections, suggesting potential design flaws or safety improvements.
Potential Accident Scenarios and AI Mitigation
- Sudden braking by a vehicle ahead: AI-powered adaptive cruise control and AEB systems can automatically adjust speed and apply brakes, thus preventing a rear-end collision. A real-world example includes vehicles equipped with radar and camera systems that are constantly monitoring the vehicle ahead, reacting to sudden braking events.
- Distracted or drowsy driving: AI systems can monitor driver behavior, identifying signs of fatigue or distraction, and prompting alerts or interventions to mitigate the risks. This might involve subtle changes in driving patterns or monitoring the driver’s eye movements.
- Adverse weather conditions: AI can analyze weather data to predict hazardous conditions and alert drivers, providing warnings or automatically adjusting vehicle systems, like adjusting tire pressure, for optimal handling.
- Pedestrian or cyclist crossings: AI-equipped vehicles can detect pedestrians and cyclists in their path, adjusting speed and applying brakes to prevent accidents. This technology is actively being developed and tested in real-world scenarios.
- Intersection collisions: AI can analyze traffic patterns at intersections to predict potential conflicts and alert drivers or even intervene autonomously to avoid collisions. This might involve adjusting the speed of the vehicle to avoid a collision or triggering a braking system.
AI in Vehicle Maintenance and Diagnostics
AI is rapidly transforming the automotive industry, and its application in vehicle maintenance and diagnostics is a significant step toward enhanced safety and efficiency. By leveraging vast datasets and sophisticated algorithms, AI systems can predict potential issues, optimize maintenance schedules, and detect safety hazards, ultimately reducing downtime and improving the overall driving experience.
Predicting Potential Vehicle Malfunctions
AI algorithms, trained on massive datasets of vehicle sensor data and maintenance records, can identify subtle patterns indicative of impending malfunctions. This predictive capability allows for proactive maintenance, preventing costly repairs and potential accidents. For instance, a car’s engine may exhibit specific sensor readings hours or even days before a significant failure. AI can detect these early warning signs and alert the owner or the service center, enabling timely intervention.
Optimizing Maintenance Schedules
AI-powered systems can analyze vehicle usage patterns, driving conditions, and sensor data to create personalized maintenance schedules. This optimized approach ensures that maintenance is performed only when necessary, reducing unnecessary costs and maximizing vehicle lifespan. Predictive maintenance, based on AI analysis, can significantly reduce the likelihood of breakdowns and ensure vehicles are always in top operational condition, enhancing safety and reliability.
Analyzing Sensor Data to Detect Potential Safety Hazards
Advanced AI systems can process data from various sensors within the vehicle, such as tire pressure, brake performance, and suspension alignment. By analyzing this real-time data, AI can detect anomalies that might indicate a potential safety hazard, such as an impending tire blowout or brake failure. Such proactive identification and alerting mechanisms contribute significantly to accident prevention. Early detection of issues, through sensor data analysis, enables timely interventions, safeguarding passengers and other road users.
AI-Powered Diagnostic Tools
AI-Powered Diagnostic Tool | Application | Benefits | Examples |
---|---|---|---|
Predictive Maintenance System | Predicts potential component failures based on usage patterns and sensor data. | Reduces unexpected downtime, minimizes repair costs, and enhances vehicle reliability. | Forecasting brake pad replacement needs, anticipating transmission fluid changes, and predicting potential engine issues. |
Real-time Sensor Monitoring System | Continuously monitors vehicle sensor data for anomalies. | Detects potential safety hazards, such as low tire pressure or brake system issues, in real time. | Alerts drivers about critical conditions, enabling immediate action. |
Automated Fault Detection System | Identifies potential malfunctions through pattern recognition in sensor data. | Reduces diagnostic time and ensures accurate fault identification. | Detects issues in the electrical system or the engine control unit (ECU) early. |
Proactive Maintenance Scheduling System | Creates personalized maintenance schedules based on vehicle usage and component performance. | Minimizes unnecessary maintenance, optimizes vehicle lifespan, and ensures optimal performance. | Recommends specific maintenance tasks at appropriate intervals. |
Ethical Considerations of AI in Car Safety
Autonomous vehicles, promising enhanced safety and efficiency, introduce complex ethical dilemmas. As AI systems become more integrated into critical decision-making processes, questions regarding responsibility, accountability, and potential bias arise. This section delves into the ethical considerations surrounding the use of AI in autonomous vehicles, emphasizing the importance of proactive measures to ensure equitable and responsible implementation.
Ethical Dilemmas in Autonomous Driving
Autonomous vehicles face scenarios where unavoidable accidents are possible. These situations require pre-programmed decision-making protocols, forcing designers to consider which values should be prioritized. For example, should the AI prioritize saving the lives of passengers over pedestrians? The lack of a universally accepted ethical framework for these scenarios poses a significant challenge. These dilemmas highlight the necessity for ongoing discussions and collaborative efforts to establish ethical guidelines for autonomous driving.
Bias in AI Systems
AI systems learn from vast datasets, and if these datasets reflect existing societal biases, the AI will likely perpetuate them. This can manifest in discriminatory outcomes, potentially affecting different demographic groups differently. For instance, if the training data for a self-driving car system predominantly features images of white drivers, the system might be less accurate in recognizing and responding to drivers of different ethnicities.
Mitigation strategies include diverse training datasets, rigorous testing protocols, and continuous monitoring of AI system outputs for potential bias.
Legal and Regulatory Challenges
The rapid advancement of AI in automotive safety presents challenges for existing legal and regulatory frameworks. Determining liability in case of accidents involving autonomous vehicles, for example, is a significant legal hurdle. Should the manufacturer, the programmer, or the vehicle owner be held responsible? Furthermore, standards and regulations for testing and certification of AI systems for automotive applications are still developing.
This highlights the need for adaptable legal and regulatory frameworks to keep pace with the technological evolution.
Real-World Ethical Concerns
Numerous real-world scenarios highlight the ethical concerns of AI in car safety. Consider the situation where an autonomous vehicle must choose between hitting a group of children or swerving into a wall, potentially injuring its passengers. The absence of a clear, universally agreed-upon ethical framework makes such scenarios highly complex. Addressing these situations requires ongoing dialogue between stakeholders, including engineers, ethicists, policymakers, and the public, to develop robust guidelines.
Data Privacy and Security in AI-Powered Cars
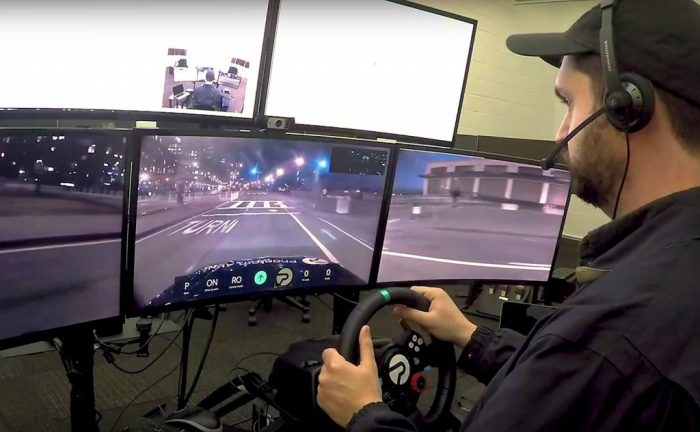
Protecting the sensitive data collected by AI systems in modern vehicles is paramount. The sheer volume of information gathered, from driving habits to environmental conditions, necessitates robust security measures to safeguard user privacy and prevent potential misuse. This crucial aspect of AI-driven car safety directly impacts the trust placed in these increasingly sophisticated technologies.
Data collected by AI systems in cars is a valuable resource, yet its inherent sensitivity demands careful handling. The information encompasses a wide range of data points, including location data, driving patterns, and even passenger behavior. Maintaining the confidentiality and integrity of this information is vital to preserve public trust and deter malicious actors.
Data Protection Mechanisms
Robust data protection mechanisms are implemented to safeguard the sensitive information collected by AI systems in cars. These measures incorporate a multi-layered approach to ensure data security and user privacy. Encryption techniques are employed to protect data both during transmission and storage. This involves converting the data into an unreadable format, thereby rendering it unusable to unauthorized individuals.
Security Measures to Prevent Hacking and Misuse
Preventing unauthorized access and malicious use of data is a top priority. A variety of security measures are employed, including robust authentication protocols, intrusion detection systems, and physical security measures. These measures are designed to detect and respond to potential threats, mitigating the risk of data breaches.
Importance of Data Privacy in AI-Driven Safety Features
Data privacy is intrinsically linked to the effectiveness and reliability of AI-driven safety features. If data breaches occur, the safety systems may become compromised, potentially jeopardizing the safety of passengers and other road users. The integrity of data is crucial for the accurate functioning of safety features, such as autonomous emergency braking or lane-keeping assistance. This integrity is ensured through rigorous data security practices.
Data Anonymization and Encryption
Data anonymization and encryption techniques play a significant role in enhancing data security. Anonymization involves removing identifying information from the data, making it impossible to trace back to a specific individual. Encryption, as mentioned earlier, transforms data into an unreadable format, further safeguarding it from unauthorized access. These techniques are employed to protect sensitive data while maintaining the value of the data for AI system training and operation.
For example, a car’s AI system can track the driver’s braking habits without linking them to a specific individual, enhancing data privacy. In another example, the encryption of location data prevents unauthorized access to real-time vehicle positions.
Public Perception and Acceptance of AI in Cars
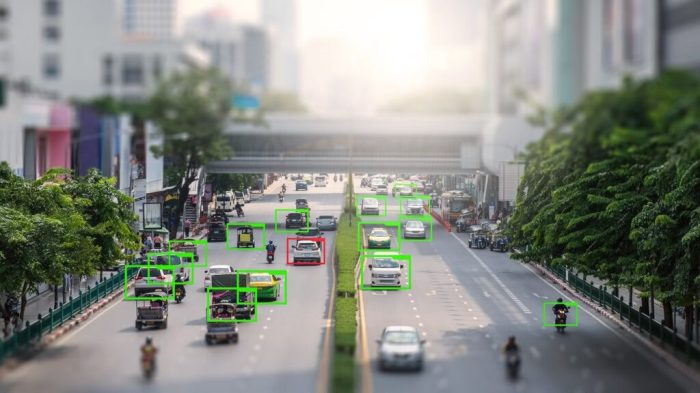
Public acceptance of AI-powered vehicles is a critical factor in their widespread adoption. Understanding public attitudes toward these vehicles and the safety features they employ is essential for successful integration into society. Public trust and confidence play a significant role in shaping the future of autonomous and semi-autonomous driving technologies.
Public perception of AI-powered vehicles is complex and multifaceted, influenced by a variety of factors. Concerns about safety, reliability, and ethical implications are frequently raised, alongside questions about the job market and the potential for misuse. Addressing these concerns proactively and transparently is crucial for fostering a positive public image and encouraging broader adoption.
Public Attitudes Toward AI-Powered Vehicles, The role of AI in car safety
Public attitudes toward AI-powered vehicles are often characterized by a mixture of excitement and apprehension. While many are intrigued by the potential benefits, such as increased safety and convenience, concerns about safety, reliability, and job displacement remain significant hurdles. Many express uncertainty about the capabilities and limitations of AI systems, particularly in unforeseen or complex situations.
Factors Influencing Public Trust and Acceptance
Several key factors influence public trust and acceptance of autonomous vehicles. Transparency in the development and deployment process, coupled with clear communication about the capabilities and limitations of AI systems, is crucial. Demonstrating a commitment to safety through rigorous testing and robust safety protocols can significantly bolster public trust. Demonstrating the reliability of the technology through real-world examples and case studies is equally important.
Furthermore, addressing public concerns about potential job displacement and ensuring equitable access to these technologies is essential for building broader acceptance.
Communication Strategies to Build Public Trust and Confidence
Effective communication strategies are vital for building public trust and confidence in AI-powered vehicles. Clear and accessible information about the technology, its benefits, and potential risks should be widely disseminated. Utilizing various communication channels, such as educational campaigns, public forums, and interactive demonstrations, can facilitate public engagement and understanding. Demonstrating a commitment to safety through rigorous testing and accident avoidance technologies can address public concerns.
Open dialogue and active listening to public concerns and feedback are crucial elements in fostering trust. Collaboration between industry experts, policymakers, and the public is essential for a successful transition to AI-powered vehicles.
Different Viewpoints on AI Adoption
“Autonomous vehicles hold the promise of revolutionizing transportation, enhancing safety, and increasing efficiency. However, the public must be involved in shaping the development and deployment process to ensure safety and accountability.”
– Dr. Emily Carter, Transportation Safety Expert
“While the technology has the potential to revolutionize the industry, we need to proceed cautiously, prioritizing safety and addressing public concerns about job displacement and ethical implications.”
– Mr. David Lee, Labor Economist
“The rapid advancement of AI in vehicles is a cause for optimism, but we must be mindful of the ethical and societal implications, and ensure that safety is paramount in the design and implementation of these systems.”
– Ms. Sophia Chen, AI Ethics Advocate
Future Trends in AI for Automotive Safety: The Role Of AI In Car Safety
The future of automotive safety hinges on the continued evolution of AI. Predictive maintenance, enhanced driver assistance, and the very concept of autonomous driving are all being reshaped by advancements in AI algorithms and data analysis. This dynamic field presents both exciting possibilities and complex challenges.
Potential Future Applications of AI in Car Safety
AI is poised to revolutionize various aspects of car safety. Beyond current driver-assistance features, we can anticipate AI-powered systems that proactively identify and mitigate potential hazards. For example, real-time analysis of traffic patterns and road conditions could enable cars to anticipate and react to potential accidents before they occur.
Advancements in AI Algorithms and Technologies
The sophistication of AI algorithms will continue to increase. Machine learning models, particularly deep learning architectures, will become more intricate, capable of processing vast amounts of data with greater accuracy and speed. This advancement will allow for more nuanced and responsive safety systems. For instance, deep learning models can be trained to recognize subtle cues in driving behavior that might indicate a driver’s fatigue or distraction, prompting proactive safety interventions.
Emerging Trends and Challenges in AI Safety Research
Several emerging trends shape the future of AI safety research. Robustness and reliability are paramount; AI systems must function consistently and predictably in a variety of scenarios, regardless of external factors like changing weather conditions or unusual driving situations. Another critical area is the development of AI systems that can adapt and learn from real-time experiences. These adaptable systems can continuously improve their performance and safety capabilities, addressing unforeseen challenges.
Future Impact of AI on the Automotive Industry
AI will fundamentally transform the automotive industry. The introduction of advanced driver-assistance systems and autonomous driving technologies will necessitate new safety standards and regulations. This shift will demand collaboration between automakers, researchers, and regulatory bodies to ensure the safe and responsible integration of AI into vehicles. Furthermore, the demand for skilled professionals in AI development and maintenance will likely increase substantially.
Companies will need to adapt their training and recruitment strategies to meet this growing demand. Examples include the development of self-driving taxi fleets, which will require a new level of safety and reliability for autonomous vehicles operating in shared public spaces.
AI-Enhanced Safety Features in Specific Vehicle Types
AI is rapidly transforming the automotive landscape, with safety improvements playing a crucial role. This evolution is not uniform across all vehicle types; the specific application of AI depends heavily on the inherent characteristics and operational requirements of each vehicle class. From optimizing braking systems in trucks to enhancing maneuverability in motorcycles, AI is adapting to the unique needs of different vehicles, leading to safer and more efficient transportation.
AI in Truck Safety
Truck drivers face unique challenges related to fatigue, visibility, and the sheer size of their vehicles. AI-powered systems can address these concerns. Advanced driver-assistance systems (ADAS) can detect potential hazards like lane departures or drowsy driving, providing warnings and even automated interventions. AI can also analyze data from sensors to anticipate potential accidents, such as identifying traffic congestion or weather patterns that may impact the road ahead.
These systems can optimize routes, monitor driver fatigue, and improve fuel efficiency, ultimately reducing the risk of accidents and improving overall operational efficiency.
AI in Motorcycle Safety
Motorcycles, with their smaller size and greater exposure to the elements, require specific safety enhancements. AI-powered systems can detect and respond to hazardous conditions more quickly than human reaction time. Real-time data analysis from sensors and cameras can anticipate sudden obstacles, enabling the motorcycle to brake or maneuver more effectively. AI can also be integrated into safety gear, such as helmets, providing real-time feedback to the rider and potentially alerting emergency services in the event of a crash.
For example, predictive algorithms can identify potential hazards based on weather conditions and traffic patterns, allowing the rider to adjust their course accordingly.
AI in Electric Car Safety
Electric vehicles (EVs) introduce new considerations for safety, particularly regarding battery management and charging infrastructure. AI can optimize battery performance and predict potential issues, minimizing the risk of fires or other critical failures. Smart charging systems, powered by AI, can optimize charging schedules to prevent overcharging or overheating, enhancing safety and efficiency. AI can also improve the safety of autonomous driving systems by processing sensor data to recognize and react to complex situations more quickly.
AI’s role in enhancing car safety is rapidly evolving, with features like autonomous braking and lane-keeping assistance becoming increasingly sophisticated. To manage the vast data streams generated by these systems, and the complex computing demands, implementing robust multi-cloud strategies Multi-cloud strategies is proving crucial. This approach allows for scalable and reliable processing, ultimately contributing to the improved safety and performance of future vehicles.
Summary Table of AI Safety Features
Vehicle Type | AI Safety Feature | Description | Example |
---|---|---|---|
Trucks | Advanced Driver-Assistance Systems (ADAS) | Systems to detect hazards, provide warnings, and potentially intervene automatically. | Lane departure warning, automatic emergency braking, adaptive cruise control. |
Motorcycles | Predictive Hazard Detection | Real-time analysis of sensor and camera data to anticipate and respond to hazards. | Anticipating sudden obstacles based on weather and traffic patterns, enabling quicker braking or maneuvering. |
Electric Cars | Optimized Battery Management | AI systems predict potential battery issues, minimizing risks of fires or critical failures. | Predicting battery overheating, optimizing charging schedules, and preventing overcharging. |
The Role of Human Interaction with AI in Safety Systems
AI-powered safety systems in vehicles are designed to enhance driver safety and reduce accidents. However, complete reliance on AI without human oversight can be problematic. Effective human interaction with these systems is crucial for maintaining a balance between automation and human control. This section delves into the critical role of human oversight and interaction within these systems.
Human oversight in AI-powered safety systems is essential to ensure safety and prevent unintended consequences. AI systems, while sophisticated, can be susceptible to errors, unexpected situations, or data limitations. Human intervention can mitigate these risks, providing a critical fail-safe mechanism. This is particularly vital in complex or unpredictable driving environments.
Importance of Human Oversight
Human oversight is paramount in maintaining control and preventing errors in complex safety systems. AI systems, while capable of processing vast amounts of data and reacting swiftly, may struggle with unforeseen circumstances or situations requiring contextual judgment. Humans possess the ability to evaluate and adapt to nuanced situations, making them critical for handling unexpected events.
Effective Human Interaction with AI Systems
Humans can interact with AI systems effectively through intuitive and well-designed interfaces. Clear communication between the human and the AI system is key. The system should provide timely and comprehensive information regarding its actions and the reasoning behind them. This transparency empowers the human driver to make informed decisions.
Design Principles for Seamless Human-AI Interaction in Critical Situations
Design principles for seamless human-AI interaction in critical situations should prioritize clarity, simplicity, and immediacy. The interface should be easily navigable and instantly responsive, allowing the driver to react quickly to emergent situations. Critical information should be presented prominently and in a format that minimizes cognitive load.
Illustrations of User Interfaces
Simple Alert System
A simple alert system would use visual cues, such as a prominent warning symbol on the instrument panel, coupled with an audible alert. The alert would provide a brief description of the potential hazard detected by the AI. This is useful for minor issues or situations requiring immediate attention but not necessarily demanding immediate human intervention.
Interactive Guidance System
An interactive guidance system would provide visual and auditory feedback to the driver. The display might include detailed explanations of the AI’s reasoning behind its recommendations and possible outcomes of different actions. This helps drivers understand the system’s decision-making process and make informed choices.
Decision Support System
A decision support system would present a range of possible responses to a critical situation, alongside a concise explanation of each option. The system could even provide a probability assessment for each outcome. This system allows the driver to evaluate different courses of action and choose the most appropriate one based on their judgment and the specific circumstances.
Last Word
In conclusion, The role of AI in car safety is poised to reshape the future of transportation. While significant advancements have been made, challenges remain in ensuring safety, ethical considerations, and public acceptance. Future trends and the integration of AI into diverse vehicle types will be key to realizing the full potential of this transformative technology. This exploration underscores the intricate interplay of technology, ethics, and societal acceptance in shaping the future of driving.
Common Queries
How does AI predict potential vehicle malfunctions?
AI analyzes vast amounts of sensor data, identifying patterns and anomalies that suggest potential mechanical issues. Machine learning algorithms can predict failures before they occur, allowing for proactive maintenance and improved safety.
What are the ethical concerns surrounding AI in autonomous vehicles?
Ethical dilemmas arise in scenarios where an autonomous vehicle must make split-second decisions, such as choosing between different potential accident outcomes. Ensuring fairness, bias mitigation, and accountability in AI systems is crucial.
How can data collected by AI systems in cars be protected?
Robust security measures, such as data encryption and anonymization, are crucial for safeguarding sensitive information. Strict protocols and regulations ensure data privacy while maintaining the integrity of AI-powered safety features.
What is the role of human interaction in AI-powered safety systems?
Humans maintain oversight in AI-powered safety systems. Effective human-AI interaction design principles are vital to ensure smooth transitions between human control and AI assistance, particularly in critical situations.